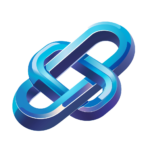
AI Integration in Clinical Decision Support Workflow Guide
AI-driven clinical decision support enhances patient care through data collection processing AI model development and ethical compliance ensuring better health outcomes
Category: AI Analytics Tools
Industry: Healthcare
AI-Driven Clinical Decision Support
1. Data Collection
1.1 Patient Data Acquisition
Collect comprehensive patient data from electronic health records (EHR), including demographics, medical history, lab results, and imaging studies.
1.2 Integration of Wearable Devices
Utilize data from wearable health devices to monitor real-time patient metrics such as heart rate, activity levels, and glucose levels.
2. Data Processing and Management
2.1 Data Cleaning and Normalization
Implement data preprocessing techniques to ensure accuracy and consistency across datasets.
2.2 Secure Data Storage
Utilize cloud-based solutions like Microsoft Azure or AWS to securely store and manage data, ensuring compliance with HIPAA regulations.
3. AI Model Development
3.1 Selection of AI Algorithms
Choose appropriate AI algorithms such as machine learning (ML) models, natural language processing (NLP), and neural networks tailored for clinical data analysis.
3.2 Training the Model
Utilize platforms like TensorFlow or PyTorch to train AI models on historical patient data, enabling them to identify patterns and predict outcomes.
4. Implementation of AI Tools
4.1 Clinical Decision Support Systems (CDSS)
Integrate AI-driven CDSS tools such as IBM Watson Health and Aidoc that provide real-time recommendations based on patient data analysis.
4.2 Risk Assessment Tools
Implement risk stratification tools like Epic’s Sepsis Prediction Model to identify patients at high risk for complications.
5. Clinical Workflow Integration
5.1 User Training and Adoption
Conduct training sessions for healthcare professionals to familiarize them with AI tools and their functionalities.
5.2 Continuous Feedback Loop
Establish a feedback mechanism to gather insights from clinicians on AI tool performance and usability.
6. Monitoring and Evaluation
6.1 Performance Metrics
Define key performance indicators (KPIs) to evaluate the effectiveness of AI-driven tools in improving clinical outcomes.
6.2 Continuous Improvement
Regularly update AI models with new data and refine algorithms to enhance accuracy and reliability.
7. Compliance and Ethical Considerations
7.1 Data Privacy and Security
Ensure adherence to data protection regulations and implement robust security measures to protect patient information.
7.2 Ethical AI Use
Establish guidelines for the ethical use of AI in healthcare, focusing on transparency, accountability, and patient consent.
Keyword: AI clinical decision support system