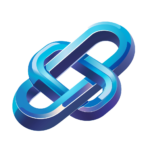
AI Integration in Drug Discovery Pipeline for Enhanced Efficiency
AI-driven drug discovery pipeline enhances efficiency by utilizing advanced algorithms for target identification compound screening and clinical trial design
Category: AI Analytics Tools
Industry: Pharmaceuticals
AI-Driven Drug Discovery Pipeline
1. Target Identification
Utilize AI algorithms to analyze biological databases and identify potential drug targets.
Tools:
- DeepMind’s AlphaFold – for protein structure prediction.
- IBM Watson – for data mining and target identification.
2. Compound Screening
Employ machine learning models to predict the efficacy of various compounds against identified targets.
Tools:
- Atomwise – uses deep learning to predict molecular interactions.
- Chemoinformatics tools (e.g., RDKit) – for virtual screening of compound libraries.
3. Preclinical Testing
Integrate AI to analyze preclinical data and optimize lead compounds based on pharmacokinetics and toxicity profiles.
Tools:
- Insilico Medicine – for drug design and preclinical predictions.
- BioSymphony – for simulating biological systems and assessing drug interactions.
4. Clinical Trial Design
Utilize predictive analytics to design efficient clinical trials, optimizing patient selection and trial protocols.
Tools:
- TrialAssure – for patient recruitment and trial optimization.
- Medidata – for data analytics and trial management.
5. Data Analysis and Biomarker Discovery
Implement AI tools to analyze clinical data and identify biomarkers for patient stratification.
Tools:
- Tempus – for genomic data analysis and biomarker identification.
- Foundation Medicine – for comprehensive genomic profiling.
6. Regulatory Submission
Leverage AI to streamline documentation and regulatory compliance processes, ensuring all necessary data is accurately presented.
Tools:
- Veeva Vault – for regulatory document management.
- ArisGlobal – for regulatory submission and compliance automation.
7. Post-Market Surveillance
Use AI analytics to monitor drug performance and patient outcomes in real-time after market launch.
Tools:
- Oracle’s Argus – for pharmacovigilance and risk management.
- HealthCatalyst – for data analytics in post-market studies.
Conclusion
The integration of AI analytics tools throughout the drug discovery pipeline enhances efficiency, reduces costs, and improves the likelihood of successful outcomes in pharmaceutical development.
Keyword: AI driven drug discovery pipeline