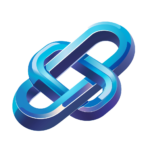
AI Powered Weather Forecasting and Risk Assessment Workflow
AI-driven weather forecasting and risk assessment enhances agriculture by utilizing real-time data predictive analytics and continuous monitoring for better decision making
Category: AI App Tools
Industry: Agriculture
Weather Forecasting and Risk Assessment
1. Data Collection
1.1 Meteorological Data
Utilize AI-driven tools such as IBM Weather Company API and Climacell to gather real-time weather data, including temperature, humidity, precipitation, and wind patterns.
1.2 Historical Weather Data
Access historical weather datasets through platforms like NOAA National Centers for Environmental Information and integrate them with AI algorithms to identify trends and patterns relevant to agricultural practices.
2. Data Processing and Analysis
2.1 Data Cleaning
Implement AI tools such as DataRobot or RapidMiner to preprocess and clean the collected data, ensuring accuracy and consistency.
2.2 Predictive Analytics
Utilize machine learning models, such as those available in Google Cloud AI or AWS SageMaker, to analyze weather data and predict future weather conditions impacting agriculture.
3. Risk Assessment
3.1 Risk Identification
Leverage AI algorithms to identify potential risks such as drought, frost, or flooding by analyzing predictive weather models and historical agricultural yield data.
3.2 Risk Evaluation
Use tools like AgriMetSoft or CropX to assess the potential impact of identified risks on crop yield and farming operations.
4. Decision Support System
4.1 Recommendations Generation
Implement AI-driven decision support systems such as FarmLogs or FieldView to provide actionable insights and recommendations for farmers based on weather forecasts and risk assessments.
4.2 Scenario Analysis
Utilize simulation tools like AgroClimate to model different agricultural scenarios based on varying weather conditions and their potential impacts on crop production.
5. Implementation and Monitoring
5.1 Strategy Implementation
Develop and execute agricultural strategies based on AI-generated insights, ensuring timely interventions to mitigate risks.
5.2 Continuous Monitoring
Employ AI tools such as Sentera or Planet Labs for ongoing monitoring of weather conditions and crop health, enabling real-time adjustments to farming practices.
6. Feedback Loop
6.1 Data Feedback
Integrate feedback mechanisms to collect data on the effectiveness of implemented strategies, using tools like AgFunder to analyze outcomes and improve future forecasts.
6.2 Continuous Improvement
Utilize insights from feedback to refine AI models and enhance the accuracy of future weather forecasting and risk assessment processes.
Keyword: AI driven weather forecasting