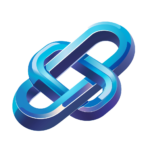
AI Powered Personalized Product Recommendation Workflow Guide
Discover an AI-driven personalized product recommendation engine that enhances customer experience through data collection processing and real-time suggestions.
Category: AI Beauty Tools
Industry: Retail
Personalized Product Recommendation Engine
1. Data Collection
1.1 Customer Data Acquisition
Gather customer information through various channels, including:
- Website registrations
- Social media interactions
- In-store purchases and loyalty programs
1.2 Product Data Compilation
Compile comprehensive product details such as:
- Ingredients
- Skin types
- Customer reviews and ratings
2. Data Processing
2.1 Data Cleaning
Utilize AI algorithms to clean and preprocess the data, ensuring accuracy and consistency.
2.2 Data Enrichment
Enhance customer profiles using external data sources, such as:
- Demographic data
- Beauty trends
3. AI Model Development
3.1 Algorithm Selection
Select appropriate AI algorithms for personalized recommendations, such as:
- Collaborative filtering
- Content-based filtering
- Deep learning models
3.2 Model Training
Train the chosen models using historical data, focusing on:
- Customer preferences
- Product performance
4. Recommendation Generation
4.1 Real-time Recommendations
Implement AI-driven tools to generate personalized product suggestions in real-time, utilizing:
- Chatbots for customer inquiries
- Recommendation engines on e-commerce platforms
4.2 User Interface Integration
Integrate recommendations into user interfaces across platforms, ensuring:
- Seamless user experience
- Accessible product information
5. Feedback Loop
5.1 Customer Feedback Collection
Gather feedback on recommendations through:
- Post-purchase surveys
- Product ratings
5.2 Continuous Improvement
Utilize feedback to refine AI models and improve accuracy, ensuring:
- Adaptation to changing customer preferences
- Enhanced recommendation quality
6. Performance Monitoring
6.1 Analytics and Reporting
Monitor the performance of the recommendation engine using KPIs such as:
- Conversion rates
- Customer satisfaction scores
6.2 Iterative Optimization
Conduct regular evaluations of the system to identify areas for enhancement and implement necessary adjustments.
Keyword: personalized product recommendation engine