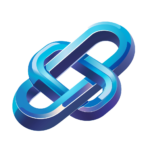
AI Powered Personalized Product Recommendations Workflow Guide
AI-driven personalized product recommendations enhance customer experiences through data collection analysis and continuous improvement for tailored shopping insights
Category: AI Business Tools
Industry: Customer Service
AI-Driven Personalized Product Recommendations
1. Data Collection
1.1 Customer Data Acquisition
Utilize customer relationship management (CRM) systems to gather customer information, including purchase history, browsing behavior, and demographic data.
1.2 Data Integration
Integrate data from multiple sources such as social media, website analytics, and transaction records to create a unified customer profile.
2. Data Analysis
2.1 Customer Segmentation
Employ AI algorithms to segment customers based on behavior patterns and preferences. Tools such as Google Analytics and Tableau can be used for this analysis.
2.2 Predictive Analytics
Utilize machine learning models to predict future purchasing behavior. Tools like IBM Watson and Microsoft Azure Machine Learning can facilitate this process.
3. Recommendation Engine Development
3.1 Algorithm Selection
Choose appropriate algorithms for generating product recommendations, such as collaborative filtering or content-based filtering.
3.2 Tool Implementation
Implement AI-driven recommendation engines like Amazon Personalize or Dynamic Yield to generate personalized product suggestions for customers.
4. User Interface Design
4.1 Integration with Customer Touchpoints
Design user interfaces for websites and mobile applications that seamlessly integrate personalized recommendations. Ensure that the UI is intuitive and enhances user experience.
4.2 A/B Testing
Conduct A/B testing to evaluate the effectiveness of different recommendation strategies using tools like Optimizely or VWO.
5. Implementation and Monitoring
5.1 Rollout
Launch the personalized recommendation system across various customer service channels, including email, chatbots, and e-commerce platforms.
5.2 Performance Monitoring
Continuously monitor the performance of the recommendation system using analytics tools. Adjust algorithms and strategies based on customer feedback and engagement metrics.
6. Continuous Improvement
6.1 Feedback Loop
Establish a feedback loop that incorporates customer insights and behavior data to refine the recommendation engine.
6.2 Iterative Updates
Regularly update the AI models and algorithms to adapt to changing customer preferences and market trends.
Keyword: AI personalized product recommendations