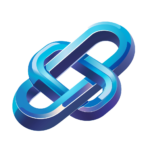
AI Powered Predictive Maintenance Workflow for Logistics Equipment
Discover how AI-driven predictive maintenance enhances logistics equipment efficiency through data collection analysis scheduling and continuous improvement
Category: AI Career Tools
Industry: Logistics and Supply Chain
Predictive Maintenance for Logistics Equipment
1. Data Collection
1.1 Identify Key Equipment
Determine which logistics equipment (e.g., forklifts, conveyor belts, trucks) will be monitored for predictive maintenance.
1.2 Gather Operational Data
Utilize IoT sensors to collect real-time data on equipment performance, including usage hours, load capacity, and environmental conditions.
1.3 Historical Maintenance Records
Compile historical maintenance data to identify patterns and common failure points.
2. Data Processing and Analysis
2.1 Data Cleaning
Ensure the collected data is accurate and formatted correctly for analysis.
2.2 Implement AI Algorithms
Utilize machine learning algorithms to analyze the data. Tools such as TensorFlow or PyTorch can be employed to build predictive models.
Example Tools:
- IBM Watson IoT Platform
- Microsoft Azure Machine Learning
2.3 Predictive Analytics
Generate predictive maintenance schedules based on the analysis, identifying when equipment is likely to fail or require maintenance.
3. Maintenance Scheduling
3.1 Automated Alerts
Set up AI-driven alerts to notify maintenance teams when equipment is approaching a predicted failure point.
3.2 Resource Allocation
Utilize AI tools to optimize resource allocation for maintenance tasks, ensuring that the right personnel and parts are available.
4. Implementation of Maintenance Actions
4.1 Execute Maintenance Tasks
Carry out the recommended maintenance actions based on predictive insights.
4.2 Continuous Monitoring
After maintenance, continue to monitor equipment performance to validate the effectiveness of the maintenance actions taken.
5. Feedback Loop and Continuous Improvement
5.1 Data Review
Regularly review the performance data post-maintenance to assess the accuracy of predictive models.
5.2 Model Refinement
Refine AI algorithms based on new data and insights to improve predictive maintenance accuracy over time.
5.3 Stakeholder Reporting
Generate reports for stakeholders to demonstrate the impact of predictive maintenance on operational efficiency and cost savings.
Keyword: predictive maintenance logistics equipment