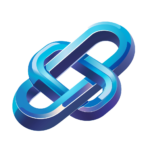
AI Driven Career Path Modeling in Pharmaceuticals Workflow
AI-driven workflow models career trajectories in the pharmaceutical industry by defining objectives collecting data and deploying predictive models for continuous improvement
Category: AI Career Tools
Industry: Pharmaceuticals
Predictive AI Career Trajectory Modeling
1. Define Objectives
1.1 Identify Key Career Paths
Determine the specific career trajectories within the pharmaceutical industry that will be modeled.
1.2 Establish Success Metrics
Define the metrics for measuring career success, such as job satisfaction, salary growth, and advancement opportunities.
2. Data Collection
2.1 Gather Historical Data
Collect historical career progression data from various sources, including employee records, industry reports, and educational backgrounds.
2.2 Integrate External Data Sources
Utilize external databases such as LinkedIn, Glassdoor, and industry publications to enrich the dataset.
3. Data Preparation
3.1 Data Cleaning
Remove inconsistencies and duplicates from the collected data to ensure accuracy.
3.2 Data Normalization
Standardize the data format for easier analysis and modeling.
4. AI Model Development
4.1 Choose AI Algorithms
Select appropriate machine learning algorithms such as regression analysis, decision trees, or neural networks for modeling career trajectories.
4.2 Implement AI Tools
Utilize AI-driven platforms like TensorFlow, IBM Watson, or Microsoft Azure Machine Learning to develop and train the predictive models.
5. Model Training and Testing
5.1 Split Data into Training and Testing Sets
Divide the dataset to train the model and validate its performance.
5.2 Evaluate Model Performance
Use metrics such as accuracy, precision, and recall to assess the effectiveness of the predictive model.
6. Deployment
6.1 Integrate with Career Tools
Embed the predictive model into existing AI career tools tailored for the pharmaceutical sector, such as career pathing applications and talent management systems.
6.2 User Training
Provide training sessions for end-users to effectively utilize the AI-driven tools.
7. Continuous Improvement
7.1 Monitor Outcomes
Regularly assess the accuracy of predictions and user satisfaction to identify areas for improvement.
7.2 Update Models
Continuously refine the predictive models based on new data and feedback to enhance their effectiveness.
8. Reporting and Insights
8.1 Generate Reports
Create detailed reports on career trajectory predictions and insights for stakeholders.
8.2 Share Findings
Disseminate findings through presentations and workshops to inform strategic decisions within the organization.
Keyword: AI career trajectory modeling