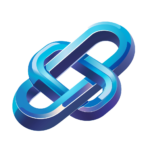
AI Driven Predictive Maintenance Scheduling System Workflow Guide
AI-driven predictive maintenance scheduling system enhances vehicle performance through real-time data collection analysis and automated scheduling for optimal efficiency
Category: AI Content Tools
Industry: Automotive
Predictive Maintenance Scheduling System
1. Data Collection
1.1 Sensor Data Acquisition
Utilize IoT sensors installed in vehicles to continuously monitor key performance indicators such as engine temperature, oil pressure, and brake wear. Tools such as PTC ThingWorx and Siemens MindSphere can be employed for real-time data gathering.
1.2 Historical Data Analysis
Aggregate historical maintenance records and performance data to identify patterns and trends. AI-driven analytics platforms like IBM Watson Analytics can be leveraged to process and analyze this data effectively.
2. Data Processing and Analysis
2.1 Predictive Analytics
Implement machine learning algorithms to analyze collected data and predict potential failures. Tools such as TensorFlow and Microsoft Azure Machine Learning can be used to develop predictive models that forecast maintenance needs.
2.2 Anomaly Detection
Utilize AI algorithms to detect anomalies in vehicle performance data that may indicate underlying issues. Solutions like DataRobot can assist in building models for real-time anomaly detection.
3. Maintenance Scheduling
3.1 Automated Scheduling
Based on predictive analytics, automate the scheduling of maintenance tasks. Tools such as UpKeep or Fiix can help in creating and managing maintenance schedules efficiently.
3.2 Resource Allocation
Optimize resource allocation by analyzing technician availability and parts inventory. AI-driven solutions like ServiceTitan can streamline this process, ensuring timely maintenance execution.
4. Execution and Monitoring
4.1 Maintenance Execution
Conduct scheduled maintenance using a mobile workforce management tool to ensure technicians have access to necessary information and resources. Tools such as Jobber can facilitate this process.
4.2 Performance Monitoring
Post-maintenance, continue to monitor vehicle performance to validate the effectiveness of maintenance activities. Utilize dashboards from tools like Tableau to visualize performance metrics and ensure ongoing compliance with maintenance standards.
5. Continuous Improvement
5.1 Feedback Loop
Establish a feedback mechanism to collect data on maintenance outcomes and technician performance. AI tools such as Qualtrics can be used to gather feedback effectively.
5.2 Model Refinement
Regularly refine predictive models based on new data and feedback to enhance accuracy. Utilize continuous learning capabilities of AI platforms like Google Cloud AI to ensure models evolve with changing conditions.
6. Reporting and Insights
6.1 Performance Reporting
Generate comprehensive reports on maintenance activities, costs, and vehicle performance. Tools such as Power BI can be integrated for detailed analytics and reporting.
6.2 Strategic Insights
Utilize insights gained from data analysis to inform strategic decision-making regarding fleet management and operational improvements. AI-driven insights can guide future investments in technology and resources.
Keyword: Predictive maintenance scheduling system