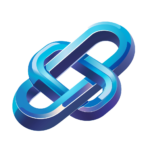
AI Integrated Nutritional Analysis and Label Generation Workflow
AI-powered nutritional analysis streamlines ingredient data collection label generation and compliance ensuring accurate and engaging food labels for consumers
Category: AI Cooking Tools
Industry: Food Packaging Industry
AI-Powered Nutritional Analysis and Label Generation
1. Data Collection
1.1 Ingredient Data Gathering
Collect comprehensive data on all ingredients used in food products, including nutritional information, sourcing details, and allergen information.
1.2 Market Research
Utilize AI-driven tools such as IBM Watson and Google Cloud AI to analyze market trends and consumer preferences related to nutritional labeling.
2. Nutritional Analysis
2.1 AI-Driven Nutritional Tools
Implement AI tools like Nutritional AI and Food Processor SQL to calculate the nutritional content of recipes based on ingredient data.
2.2 Validation and Accuracy Check
Use machine learning algorithms to cross-verify nutritional data against established databases such as USDA FoodData Central.
3. Label Generation
3.1 Automated Label Design
Employ AI-based design software like Canva Pro or Adobe Spark to create visually appealing labels that comply with regulatory requirements.
3.2 Dynamic Content Integration
Integrate dynamic content generation tools such as GPT-3 for crafting engaging product descriptions and usage suggestions based on nutritional analysis.
4. Compliance and Quality Assurance
4.1 Regulatory Compliance Check
Implement AI systems to ensure that labels meet local and international food labeling regulations, such as Label Insight.
4.2 Continuous Monitoring
Utilize AI for ongoing monitoring of compliance and quality, allowing for real-time updates in labeling as regulations change.
5. Distribution and Feedback
5.1 Distribution of Labels
Use cloud-based solutions like Microsoft Azure for efficient distribution of digital labels to packaging lines.
5.2 Consumer Feedback Analysis
Leverage sentiment analysis tools powered by AI, such as MonkeyLearn, to gather and analyze consumer feedback on packaging and labeling.
6. Iteration and Improvement
6.1 Data-Driven Insights
Analyze collected consumer feedback and sales data to refine nutritional offerings and labeling strategies.
6.2 Continuous Learning
Implement machine learning models to continuously improve the accuracy of nutritional analysis and labeling based on new data inputs.
Keyword: AI nutritional analysis tools