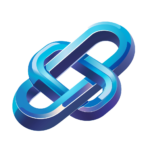
AI Integration in Fraud Detection Workflow for Claims Management
AI-driven workflow enhances fraud detection in claims through data preprocessing machine learning analysis risk assessment and streamlined communication with customers
Category: AI Customer Service Tools
Industry: Insurance
AI-Assisted Fraud Detection in Claims
1. Initial Claim Submission
1.1 Customer Interaction
Customers submit claims through various channels, including web portals, mobile apps, and customer service representatives.
1.2 Data Collection
Collect relevant information such as claim details, customer information, and supporting documents.
2. Data Preprocessing
2.1 Data Cleaning
Utilize AI-powered tools such as DataRobot or Trifacta to clean and preprocess the data for analysis.
2.2 Feature Extraction
Identify key features that may indicate fraudulent activity, such as claim amounts, frequency of claims, and historical data.
3. Fraud Detection Analysis
3.1 Machine Learning Model Training
Implement machine learning algorithms using platforms like TensorFlow or Scikit-learn to train models on historical claims data.
3.2 Anomaly Detection
Use AI-driven tools such as IBM Watson or SAS Fraud Management to identify anomalies in claims that deviate from established patterns.
4. Risk Assessment
4.1 Risk Scoring
Assign risk scores to claims based on the outputs of the fraud detection models, categorizing them as low, medium, or high risk.
4.2 Automated Alerts
Set up automated alerts for high-risk claims using AI systems to notify claims adjusters for further investigation.
5. Investigation Process
5.1 Claims Adjuster Review
Claims adjusters review flagged claims, utilizing AI tools like ClaimVantage for streamlined case management.
5.2 Additional Data Gathering
Leverage AI to gather additional data from external sources, such as social media and public records, to support the investigation.
6. Decision Making
6.1 Approval or Denial
Based on the investigation findings, claims are either approved or denied. AI tools can assist in documenting the rationale for decisions.
6.2 Feedback Loop
Incorporate feedback from the decision-making process to continuously improve the fraud detection models using machine learning techniques.
7. Reporting and Analytics
7.1 Performance Metrics
Utilize AI analytics tools like Tableau or Power BI to generate reports on fraud detection performance and trends.
7.2 Continuous Improvement
Regularly update machine learning models and algorithms based on new data and insights to enhance fraud detection capabilities.
8. Customer Communication
8.1 Notification of Claim Status
Use AI chatbots, such as Zendesk Chat or Intercom, to communicate claim status updates to customers promptly.
8.2 Customer Feedback Collection
Gather customer feedback on the claims process using AI-driven survey tools to identify areas for improvement.
Keyword: AI fraud detection workflow