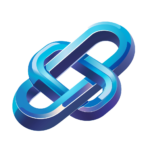
AI Driven Precision Medicine Workflow for Personalized Treatment
Discover how AI-driven workflows enhance precision medicine through personalized treatment planning data integration and continuous monitoring for improved healthcare outcomes
Category: AI Data Tools
Industry: Healthcare
Precision Medicine and Personalized Treatment Planning
1. Data Collection
1.1 Patient Data Acquisition
Utilize electronic health records (EHR) to gather comprehensive patient data including demographics, medical history, and genetic information.
1.2 Wearable Devices and Mobile Health Applications
Incorporate data from wearable devices and mobile health applications to monitor real-time health metrics.
2. Data Integration and Management
2.1 Centralized Data Repository
Establish a centralized database to integrate diverse data sources, ensuring data standardization and accessibility.
2.2 AI-Driven Data Cleaning Tools
Implement AI-driven tools such as Trifacta or Talend for data cleaning and preprocessing to enhance data quality.
3. Data Analysis
3.1 Predictive Analytics
Utilize AI algorithms to analyze patient data and predict health outcomes. Tools like IBM Watson Health can provide insights into disease progression.
3.2 Genomic Data Analysis
Employ genomic analysis tools such as Illumina BaseSpace or GRAIL to interpret genetic data and identify potential treatment options.
4. Treatment Planning
4.1 Personalized Treatment Recommendations
Leverage AI systems like Tempus or Foundation Medicine to generate personalized treatment plans based on individual patient profiles.
4.2 Clinical Decision Support Systems (CDSS)
Implement CDSS tools such as UpToDate or ClinicalKey to assist healthcare providers in making informed treatment decisions.
5. Implementation and Monitoring
5.1 Treatment Execution
Initiate the personalized treatment plan, ensuring all healthcare providers are aligned with the strategy.
5.2 Continuous Monitoring and Feedback
Utilize AI-driven monitoring tools like Biofourmis or Health Catalyst to track patient progress and adjust treatment plans as necessary.
6. Outcome Evaluation
6.1 Performance Metrics Analysis
Analyze treatment outcomes using AI analytics platforms to evaluate the effectiveness of personalized treatment plans.
6.2 Patient Feedback Collection
Gather patient feedback through surveys and interviews to assess satisfaction and areas for improvement.
7. Iterative Improvement
7.1 Data-Driven Refinement
Utilize insights gained from outcome evaluations to refine data collection, analysis, and treatment planning processes.
7.2 Continuous Learning and Adaptation
Implement machine learning algorithms that learn from new data to continuously improve treatment recommendations and healthcare outcomes.
Keyword: personalized treatment planning AI