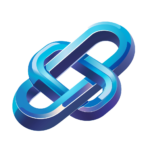
AI Integration in Drug Discovery Pipeline for Enhanced Outcomes
AI-driven drug discovery enhances efficiency from target identification to post-market surveillance improving outcomes in pharmaceutical development
Category: AI Data Tools
Industry: Pharmaceuticals
AI-Driven Drug Discovery Pipeline
1. Target Identification
Utilize AI algorithms to analyze biological data and identify potential drug targets.
- Tools:
- DeepMind’s AlphaFold for protein structure prediction
- IBM Watson for Genomics to analyze genomic data
2. Compound Screening
Employ machine learning models to predict the efficacy of compounds against identified targets.
- Tools:
- Schrödinger Suite for virtual screening
- Atomwise’s AI-driven drug discovery platform for high-throughput screening
3. Lead Optimization
Leverage AI to optimize lead compounds by predicting their pharmacokinetic properties and potential toxicity.
- Tools:
- ChemAxon for cheminformatics and molecular modeling
- Insilico Medicine’s PandaOmics for drug design and optimization
4. Preclinical Testing
Utilize AI to analyze data from preclinical studies and predict outcomes in clinical trials.
- Tools:
- BioSymetrics for predictive analytics in preclinical testing
- GNS Healthcare for modeling patient responses to treatments
5. Clinical Trial Design
Implement AI to optimize clinical trial designs, including patient selection and endpoint determination.
- Tools:
- Tempus for data-driven clinical trial design
- TrialSpark for AI-enhanced patient recruitment
6. Post-Market Surveillance
Employ AI tools for monitoring drug performance and safety post-launch.
- Tools:
- Oracle’s Argus for pharmacovigilance
- IBM Watson for analyzing real-world evidence and adverse events
Conclusion
The integration of AI tools throughout the drug discovery pipeline enhances efficiency, reduces time-to-market, and improves the likelihood of successful outcomes in pharmaceutical development.
Keyword: AI drug discovery pipeline