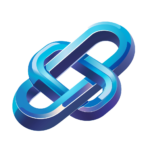
AI Driven Automated Network Troubleshooting Workflow for Efficiency
Discover AI-driven automated network troubleshooting that enhances issue detection data collection root cause analysis and remediation for optimal performance
Category: AI Data Tools
Industry: Telecommunications
Automated Network Troubleshooting
1. Issue Detection
1.1 Network Monitoring Tools
Utilize AI-driven network monitoring tools such as SolarWinds Network Performance Monitor or Palo Alto Networks Prisma to continuously analyze network traffic and identify anomalies.
1.2 Anomaly Detection Algorithms
Implement machine learning algorithms to detect unusual patterns in network performance, such as spikes in latency or packet loss, indicating potential issues.
2. Data Collection
2.1 Automated Data Gathering
Leverage tools like Splunk or Datadog to automatically collect logs and performance metrics from network devices.
2.2 Centralized Data Repository
Store collected data in a centralized repository such as Amazon S3 or Google Cloud Storage for easier analysis and access.
3. Root Cause Analysis
3.1 AI-Powered Analysis Tools
Utilize AI-driven analysis tools like IBM Watson AIOps or Moogsoft to correlate data and identify the root cause of network issues efficiently.
3.2 Predictive Analytics
Apply predictive analytics to foresee potential network failures before they occur, using tools such as Microsoft Azure Machine Learning.
4. Automated Remediation
4.1 AI-Based Remediation Systems
Implement automated remediation systems that utilize AI, such as ServiceNow, to execute predefined scripts or workflows that resolve identified issues without human intervention.
4.2 Feedback Loop
Establish a feedback loop where the system learns from past incidents to improve future troubleshooting processes, enhancing the accuracy of AI predictions.
5. Reporting and Continuous Improvement
5.1 Automated Reporting Tools
Use reporting tools like Tableau or Power BI to generate automated reports on network performance and troubleshooting outcomes.
5.2 Continuous Learning
Integrate continuous learning mechanisms to refine AI models based on new data and evolving network conditions, ensuring ongoing optimization of troubleshooting processes.
Keyword: AI driven network troubleshooting