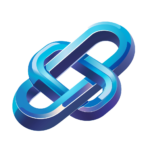
Automated Quality Control in 3D Printing with AI Integration
Discover how AI-driven workflows enhance quality control and error detection in 3D printing from design to post-printing analysis for optimal results
Category: AI Design Tools
Industry: 3D Printing and Prototyping
Automated Quality Control and Error Detection in 3D Printing
1. Initial Design Phase
1.1 Concept Development
Utilize AI-driven design tools such as Autodesk’s Fusion 360 or SolidWorks to create initial 3D models. These tools incorporate generative design algorithms to optimize the design for functionality and manufacturability.
1.2 Design Validation
Implement AI-based simulation software like ANSYS or SimScale to validate the design under real-world conditions. This step helps identify potential structural weaknesses or design flaws before moving to the prototyping phase.
2. Pre-Printing Quality Assurance
2.1 Model Repair and Optimization
Use tools like Meshmixer or Netfabb to automatically repair any mesh errors in the design. These AI tools can detect and correct issues such as non-manifold edges or holes in the model.
2.2 Slicing and Toolpath Generation
Employ slicing software with integrated AI capabilities, such as Ultimaker Cura or PrusaSlicer, to generate optimized toolpaths. These tools analyze the model and adjust settings for optimal print quality and material usage.
3. Printing Phase
3.1 Real-Time Monitoring
Integrate AI-powered monitoring systems, such as 3DPrinterOS or OctoPrint with AI plugins, to oversee the printing process. These systems can detect anomalies in real-time, such as layer misalignment or filament jams.
3.2 Automated Error Detection
Utilize machine learning algorithms to analyze data from sensors and cameras during printing. For instance, systems like PrintScan can provide immediate feedback on print quality and suggest corrective actions.
4. Post-Printing Quality Control
4.1 Dimensional Inspection
Implement AI-based inspection tools, such as GOM Inspect or Zeiss T-SCAN, to perform dimensional analysis of the printed parts. These tools can automatically compare the printed object against the original design specifications.
4.2 Surface Quality Assessment
Use AI-driven image recognition software, like Deep Learning-based vision systems, to assess the surface finish of the printed parts. This can identify defects such as layer separation or surface roughness.
5. Feedback Loop and Continuous Improvement
5.1 Data Collection and Analysis
Gather data from all stages of the workflow, including design iterations, printing parameters, and quality assessments. Use AI analytics tools to identify trends and areas for improvement.
5.2 Iterative Design Enhancement
Leverage insights gained from data analysis to refine the design and printing process. Tools like Siemens NX can facilitate rapid prototyping and adjustments based on feedback from previous prints.
6. Documentation and Reporting
6.1 Automated Reporting
Generate comprehensive reports using AI-driven documentation tools that compile data from the entire workflow. This ensures that all quality control measures and outcomes are well-documented for future reference.
6.2 Compliance and Certification
Ensure that all quality control processes are compliant with industry standards. Use AI tools to streamline the certification process, ensuring that all printed parts meet regulatory requirements.
Keyword: AI driven quality control 3D printing