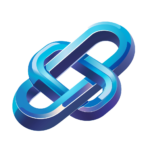
AI-Driven Workflow for Quality of Service Monitoring and Improvement
AI-driven QoS monitoring enhances service quality by defining metrics collecting data analyzing insights and implementing improvement strategies for optimal performance
Category: AI Domain Tools
Industry: Telecommunications
AI-Driven Quality of Service (QoS) Monitoring and Improvement
1. Define Quality of Service Metrics
1.1 Identify Key Performance Indicators (KPIs)
Establish metrics such as latency, jitter, packet loss, and throughput that are critical for assessing service quality.
1.2 Set Baseline Performance Levels
Determine acceptable performance thresholds for each KPI based on historical data and industry standards.
2. Data Collection
2.1 Implement AI-Driven Monitoring Tools
Utilize tools such as:
- NetOps AI: For real-time network performance monitoring.
- IBM Watson: To analyze large datasets for QoS insights.
- SolarWinds: For comprehensive network performance management.
2.2 Gather Data from Various Sources
Collect data from network devices, user feedback, and external sources such as social media to gain a holistic view of service quality.
3. Data Analysis
3.1 Utilize AI Algorithms for Data Processing
Employ machine learning algorithms to identify patterns and anomalies in the collected data.
3.2 Generate Insights and Reports
Use AI-driven analytics tools like:
- Google Cloud AI: For predictive analytics on QoS trends.
- Microsoft Azure AI: To visualize data and derive actionable insights.
4. Identify Areas for Improvement
4.1 Conduct Root Cause Analysis
Leverage AI tools to perform root cause analysis on identified issues affecting QoS.
4.2 Prioritize Issues Based on Impact
Rank issues based on their effect on user experience and operational efficiency.
5. Implement Improvement Strategies
5.1 Develop Action Plans
Create detailed plans to address the prioritized issues, including timelines and responsible teams.
5.2 Utilize AI-Driven Solutions for Implementation
Incorporate tools such as:
- Cisco AI Network Analytics: For automated network adjustments.
- Juniper Mist: To enhance Wi-Fi performance through AI-driven insights.
6. Continuous Monitoring and Feedback Loop
6.1 Establish a Feedback Mechanism
Set up channels for user feedback to continuously assess service quality post-implementation.
6.2 Iterate on Improvement Strategies
Regularly review performance data and user feedback to refine and enhance QoS strategies using AI tools.
7. Reporting and Stakeholder Engagement
7.1 Generate Regular Reports
Provide stakeholders with comprehensive reports on QoS performance and improvement outcomes.
7.2 Engage Stakeholders for Insights
Conduct meetings with stakeholders to discuss findings and gather additional insights for future improvements.
Keyword: AI-driven QoS monitoring strategies