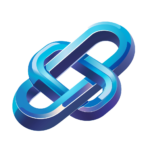
AI Driven Predictive Analytics Workflow for Portfolio Management
AI-driven predictive analytics enhances portfolio management through data collection preprocessing model development and real-time insights for strategic decisions
Category: AI Finance Tools
Industry: Insurance
Predictive Analytics for Portfolio Management
1. Data Collection
1.1 Identify Data Sources
Gather data from various sources including:
- Internal databases (claims, policyholder information)
- External market data (economic indicators, competitor analysis)
- Social media and news sentiment analysis
1.2 Data Aggregation
Utilize ETL (Extract, Transform, Load) tools to consolidate data into a centralized repository.
- Tools: Apache NiFi, Talend
2. Data Preprocessing
2.1 Data Cleaning
Remove inconsistencies and fill in missing values to ensure data quality.
2.2 Data Normalization
Standardize data formats and scales for effective analysis.
3. Feature Engineering
3.1 Identify Key Features
Determine which variables have the most predictive power for portfolio performance.
3.2 Create New Features
Generate additional features through techniques such as:
- Time-series analysis
- Sentiment scoring from textual data
4. Model Development
4.1 Select AI Algorithms
Choose appropriate machine learning algorithms based on the data characteristics.
- Regression models (e.g., Linear Regression, Lasso)
- Tree-based models (e.g., Random Forest, XGBoost)
4.2 Model Training
Train models using historical data to predict future portfolio performance.
- Tools: TensorFlow, Scikit-learn
5. Model Evaluation
5.1 Performance Metrics
Assess model accuracy using metrics such as:
- Mean Absolute Error (MAE)
- Root Mean Square Error (RMSE)
5.2 Cross-Validation
Implement k-fold cross-validation to ensure the model’s robustness.
6. Implementation
6.1 Deploying the Model
Integrate the predictive model into the existing portfolio management system.
- Tools: AWS SageMaker, Microsoft Azure ML
6.2 Real-time Monitoring
Set up dashboards for continuous monitoring of model performance and portfolio metrics.
- Tools: Tableau, Power BI
7. Insights Generation
7.1 Automated Reporting
Generate reports that summarize predictive insights and portfolio recommendations.
7.2 Decision Support
Utilize AI-driven insights to inform strategic investment decisions.
8. Feedback Loop
8.1 Model Retraining
Regularly update the model with new data to improve accuracy and adapt to market changes.
8.2 Continuous Improvement
Solicit feedback from stakeholders to refine the predictive analytics process.
Keyword: Predictive analytics for portfolio management