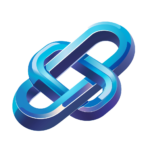
AI Driven Revenue Forecasting and Optimization Workflow Guide
AI-driven revenue forecasting optimizes financial strategies through data collection analysis model development and continuous monitoring for improved accuracy and insights
Category: AI Finance Tools
Industry: Telecommunications
AI-Driven Revenue Forecasting and Optimization
1. Data Collection
1.1 Identify Data Sources
Gather historical revenue data, customer demographics, market trends, and competitive analysis from various sources.
1.2 Data Integration
Utilize ETL (Extract, Transform, Load) tools such as Talend or Apache Nifi to consolidate data from disparate sources into a centralized data warehouse.
2. Data Preparation
2.1 Data Cleansing
Employ data cleansing techniques to remove inaccuracies and inconsistencies in the dataset using tools like OpenRefine or Trifacta.
2.2 Feature Engineering
Develop relevant features that can impact revenue forecasting, such as seasonal trends and customer behavior patterns.
3. AI Model Development
3.1 Select AI Algorithms
Choose appropriate algorithms for revenue forecasting, such as time series analysis, regression models, or neural networks.
3.2 Tool Selection
Utilize AI-driven platforms like TensorFlow or H2O.ai for building and training forecasting models.
3.3 Model Training
Train the selected models using historical data, ensuring to validate the models with a separate test dataset.
4. Revenue Forecasting
4.1 Generate Forecasts
Utilize the trained models to generate revenue forecasts for different time frames (monthly, quarterly, annually).
4.2 Scenario Analysis
Conduct scenario analysis to evaluate the impact of various market conditions on revenue forecasts using tools like What-If Analysis in Excel or IBM Planning Analytics.
5. Optimization Strategies
5.1 Identify Optimization Opportunities
Analyze forecast results to identify areas for revenue optimization, such as pricing strategies or promotional campaigns.
5.2 Implement AI-Driven Recommendations
Use AI tools like Salesforce Einstein or Microsoft Azure Machine Learning to implement and monitor optimization strategies.
6. Monitoring and Adjustment
6.1 Continuous Monitoring
Set up dashboards using BI tools like Tableau or Power BI to continuously monitor revenue performance against forecasts.
6.2 Model Refinement
Regularly refine forecasting models based on new data and market changes to improve accuracy.
7. Reporting and Insights
7.1 Generate Reports
Create comprehensive reports summarizing forecasts, optimization strategies, and performance metrics for stakeholders.
7.2 Strategic Recommendations
Provide actionable insights and recommendations to executive teams based on forecasted data and optimization outcomes.
Keyword: AI-driven revenue forecasting strategies