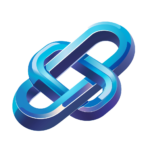
AI Driven Predictive Analytics for Effective Trial Site Selection
AI-driven predictive analytics optimizes trial site selection by defining objectives gathering data and implementing models for improved performance and decision making
Category: AI Health Tools
Industry: Clinical trial management companies
Predictive Analytics for Trial Site Selection
1. Define Objectives
1.1 Identify Key Performance Indicators (KPIs)
Determine metrics that will measure the success of trial site selection, such as patient recruitment rates, site engagement levels, and historical performance data.
1.2 Establish Target Population
Define the demographic and geographic characteristics of the patient population relevant to the clinical trial.
2. Data Collection
2.1 Gather Historical Data
Collect data from previous clinical trials, including site performance metrics, patient demographics, and recruitment timelines.
2.2 Integrate External Data Sources
Utilize external datasets such as electronic health records (EHRs), social determinants of health, and regional healthcare statistics.
3. Data Preprocessing
3.1 Clean and Normalize Data
Ensure data accuracy by removing duplicates, correcting errors, and standardizing formats.
3.2 Feature Engineering
Create relevant features that can enhance predictive modeling, such as site-specific characteristics and patient eligibility criteria.
4. Implement AI-Driven Predictive Models
4.1 Select Appropriate AI Tools
Utilize AI-driven platforms such as IBM Watson for Clinical Trials, Medidata Solutions, or Oracle’s Siebel Clinical to build predictive models.
4.2 Train Predictive Models
Use machine learning algorithms to analyze historical data and predict site performance. Techniques may include regression analysis, decision trees, and neural networks.
5. Site Selection Analysis
5.1 Evaluate Predictive Outcomes
Assess the predictive analytics results to identify the most suitable trial sites based on the defined KPIs.
5.2 Rank Potential Sites
Rank the sites based on predicted performance metrics, patient accessibility, and historical success rates.
6. Decision Making
6.1 Collaborate with Stakeholders
Engage with clinical operations, regulatory affairs, and site management teams to discuss the findings and finalize site selection.
6.2 Document Rationale for Site Selection
Provide a comprehensive report detailing the predictive analytics process, chosen sites, and justification for decisions made.
7. Continuous Monitoring and Feedback
7.1 Track Site Performance
Monitor the performance of selected sites throughout the trial, using real-time data analytics tools to ensure alignment with predictions.
7.2 Refine Models Based on Outcomes
Adjust predictive models based on actual site performance and recruitment data to improve future trial site selection processes.
8. Reporting and Insights
8.1 Generate Comprehensive Reports
Create detailed reports summarizing the predictive analytics process, site selection outcomes, and lessons learned.
8.2 Share Insights with the Broader Team
Disseminate findings and insights across the organization to enhance future clinical trial strategies and site selection methodologies.
Keyword: predictive analytics trial site selection