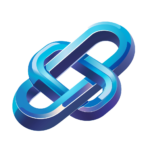
AI Integration in Biomarker Discovery and Validation Workflow
AI-driven biomarker discovery enhances research through multidisciplinary teams data integration and predictive modeling ensuring robust validation and regulatory compliance
Category: AI Health Tools
Industry: Medical research institutions
AI-Assisted Biomarker Discovery and Validation
1. Identification of Research Objectives
1.1 Define Research Goals
Establish specific objectives for biomarker discovery, such as disease type, target population, and desired outcomes.
1.2 Assemble a Multidisciplinary Team
Form a team of researchers, clinicians, data scientists, and bioinformaticians to ensure a comprehensive approach.
2. Data Collection
2.1 Gather Relevant Data
Collect clinical data, genomic data, proteomic data, and other omics data relevant to the research objectives.
2.2 Utilize AI Tools for Data Integration
Implement AI-driven platforms such as IBM Watson or Google Cloud AutoML to integrate diverse datasets for a holistic view.
3. Data Preprocessing
3.1 Clean and Normalize Data
Use AI algorithms to identify and rectify inconsistencies, missing values, and outliers in the dataset.
3.2 Feature Selection
Employ machine learning techniques, such as Random Forest and LASSO regression, to select the most relevant features for biomarker identification.
4. Biomarker Discovery
4.1 Implement Machine Learning Models
Utilize supervised and unsupervised learning algorithms to analyze the data. Examples include:
- Support Vector Machines (SVM) for classification tasks.
- Deep Learning frameworks like TensorFlow or Keras for complex pattern recognition.
4.2 Validate Initial Findings
Cross-validate results using techniques such as k-fold cross-validation to ensure robustness and reliability.
5. Biomarker Validation
5.1 Conduct Experimental Validation
Design and execute laboratory experiments to validate the identified biomarkers using techniques like ELISA or qPCR.
5.2 Utilize AI for Predictive Modeling
Apply predictive analytics tools such as Azure Machine Learning to forecast the clinical utility of biomarkers.
6. Clinical Trial Design
6.1 Develop Clinical Trial Protocols
Create protocols that integrate the validated biomarkers into clinical settings for further evaluation.
6.2 Leverage AI for Patient Recruitment
Use AI-driven platforms like TrialX to identify and recruit suitable patient populations for clinical trials.
7. Regulatory Submission
7.1 Prepare Documentation
Compile data and findings into a comprehensive submission package for regulatory bodies.
7.2 Utilize AI Tools for Compliance Checking
Employ AI solutions such as Veeva Vault to ensure all documentation meets regulatory standards.
8. Post-Market Surveillance
8.1 Monitor Biomarker Performance
Continuously assess the effectiveness and safety of biomarkers in real-world settings using AI analytics tools.
8.2 Implement Feedback Mechanisms
Utilize AI to analyze feedback from clinical practitioners and patients to refine biomarker applications.
Keyword: AI biomarker discovery process