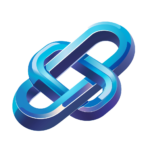
Personalized Energy Saving Workflow with AI Integration
Discover personalized energy-saving recommendations through an AI-driven workflow that analyzes user data and optimizes energy consumption for smarter living
Category: AI Home Tools
Industry: Energy Management
Personalized Energy-Saving Recommendations Workflow
1. Data Collection
1.1 User Profile Setup
Gather essential information from the user, including household size, energy usage patterns, and preferences. This can be facilitated through an intuitive onboarding questionnaire in an AI-driven app.
1.2 Smart Device Integration
Integrate with existing smart home devices such as thermostats (e.g., Nest, Ecobee), smart plugs (e.g., TP-Link Kasa), and energy monitors (e.g., Sense). This allows for real-time data collection on energy consumption.
2. Data Analysis
2.1 AI-Driven Insights
Utilize machine learning algorithms to analyze collected data. For example, tools like Google Cloud AI can process usage patterns and identify inefficiencies.
2.2 Benchmarking
Compare user data against industry standards and similar households to identify potential energy-saving opportunities.
3. Recommendation Generation
3.1 Personalized Suggestions
Generate tailored recommendations based on data analysis. For instance, suggest optimal thermostat settings or the best times to run high-energy appliances.
3.2 AI-Driven Tools
Incorporate tools like EnergyHub or Sense Energy to provide users with actionable insights and real-time notifications about their energy consumption.
4. Implementation Support
4.1 User Guidance
Offer step-by-step guidance for users to implement the recommendations. This can be delivered through the app or via smart home assistants like Amazon Alexa or Google Assistant.
4.2 Progress Tracking
Enable users to track their energy savings over time using visual dashboards that display consumption trends and savings achieved.
5. Feedback Loop
5.1 User Feedback Collection
Solicit user feedback on the effectiveness of the recommendations and overall satisfaction through surveys or app prompts.
5.2 Continuous Improvement
Utilize feedback to refine algorithms and improve the personalization of future recommendations, ensuring the system evolves with user needs.
6. Reporting
6.1 Monthly Energy Reports
Provide users with monthly reports summarizing their energy usage, savings achieved, and further recommendations for improvement.
6.2 AI-Enhanced Analytics
Implement AI tools like IBM Watson to analyze long-term trends and provide insights for future energy management strategies.
Keyword: personalized energy saving recommendations