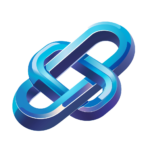
AI Integration in Medical Imaging Quality Assurance Workflow
AI-driven quality assurance in medical imaging enhances diagnosis through automated image capture noise reduction and seamless integration with healthcare systems
Category: AI Image Tools
Industry: Healthcare
AI-Driven Quality Assurance in Medical Imaging
1. Initial Image Acquisition
1.1 Selection of Imaging Modality
Choose the appropriate imaging modality (e.g., MRI, CT, X-ray) based on clinical requirements.
1.2 Image Capture
Utilize advanced imaging devices to capture high-resolution images, ensuring optimal conditions for image quality.
2. Pre-Processing of Images
2.1 Noise Reduction
Implement AI algorithms such as convolutional neural networks (CNNs) for noise reduction and enhancement of image clarity.
2.2 Image Normalization
Apply AI-driven tools like ImageJ or ITK-SNAP to standardize image formats and intensities across different modalities.
3. Quality Assessment
3.1 Automated Quality Control
Utilize AI-powered platforms such as Zebra Medical Vision or Aidoc to automatically assess image quality and flag any anomalies.
3.2 Human Review
Incorporate radiologist expertise to review flagged images, ensuring a combination of AI efficiency and human intuition.
4. Diagnosis Support
4.1 AI-Enhanced Diagnosis
Leverage tools like Google Health’s AI algorithms to assist radiologists in diagnosing conditions based on imaging data.
4.2 Reporting
Utilize AI-driven reporting tools such as Qure.ai to generate standardized reports that highlight key findings and recommendations.
5. Continuous Learning and Improvement
5.1 Feedback Loop
Establish a feedback mechanism where radiologists provide insights on AI performance, allowing for continuous model improvement.
5.2 Data Collection for Model Training
Gather and anonymize imaging data to enhance AI algorithms, ensuring they are trained on diverse datasets for improved accuracy.
6. Implementation and Integration
6.1 System Integration
Integrate AI tools with existing healthcare IT systems (e.g., PACS, EHR) for seamless workflow and data exchange.
6.2 Staff Training
Conduct training sessions for healthcare professionals to familiarize them with AI tools and their applications in quality assurance.
7. Evaluation and Compliance
7.1 Performance Metrics
Establish key performance indicators (KPIs) to evaluate the effectiveness of AI-driven quality assurance processes.
7.2 Regulatory Compliance
Ensure compliance with healthcare regulations (e.g., HIPAA, FDA) when deploying AI tools in medical imaging.
Keyword: AI quality assurance medical imaging