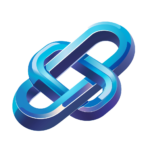
Optimize Predictive Maintenance with AI Integration Workflow
Discover how AI-driven predictive maintenance optimizes operations through real-time data collection analytics and proactive maintenance scheduling
Category: AI Networking Tools
Industry: Manufacturing
Predictive Maintenance Optimization
1. Data Collection
1.1 Sensor Integration
Utilize IoT sensors to gather real-time data from machinery, including temperature, vibration, and operational speed.
1.2 Historical Data Analysis
Aggregate historical maintenance records and operational data to identify patterns and trends in equipment performance.
2. Data Processing
2.1 Data Cleaning
Implement data preprocessing techniques to filter out noise and irrelevant information from the collected data.
2.2 Data Storage
Utilize cloud-based storage solutions such as AWS S3 or Google Cloud Storage to securely store large volumes of data.
3. Predictive Analytics
3.1 AI Model Development
Develop machine learning models using tools such as TensorFlow or PyTorch to predict potential equipment failures based on historical and real-time data.
3.2 Model Training
Train the models on historical data sets to enhance accuracy, using techniques like supervised learning for anomaly detection.
4. Implementation of Predictive Maintenance
4.1 Real-Time Monitoring
Deploy AI-driven analytics platforms like IBM Watson IoT or Siemens MindSphere to monitor equipment health in real-time.
4.2 Maintenance Scheduling
Utilize predictive insights to schedule maintenance activities proactively, reducing downtime and extending equipment lifespan.
5. Continuous Improvement
5.1 Performance Evaluation
Regularly assess the effectiveness of predictive maintenance strategies through key performance indicators (KPIs) such as maintenance costs and equipment uptime.
5.2 Feedback Loop
Incorporate feedback from maintenance teams to refine AI models and improve predictive accuracy over time.
6. Reporting and Documentation
6.1 Automated Reporting
Utilize business intelligence tools like Tableau or Power BI to generate automated reports on maintenance activities and predictive analytics outcomes.
6.2 Documentation of Best Practices
Document successful strategies and lessons learned to foster a culture of continuous improvement in predictive maintenance.
7. Stakeholder Engagement
7.1 Training and Development
Provide training sessions for staff on the use of AI tools and the importance of predictive maintenance in enhancing operational efficiency.
7.2 Stakeholder Communication
Regularly communicate the benefits and successes of predictive maintenance initiatives to all stakeholders, ensuring alignment and support.
Keyword: Predictive maintenance optimization strategies