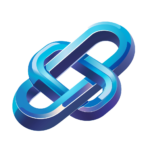
Real Time Quality of Service Management with AI Integration
AI-driven real-time quality of service management enhances network performance through data collection analysis monitoring automation and continuous improvement.
Category: AI Networking Tools
Industry: Telecommunications
Real-Time Quality of Service Management
1. Workflow Overview
This workflow outlines the process for managing Quality of Service (QoS) in real-time using AI networking tools within the telecommunications sector. The integration of artificial intelligence enhances the monitoring, analysis, and optimization of network performance.
2. Stakeholders
- Network Operations Team
- AI Development Team
- Customer Support Team
- End Users
3. Workflow Steps
Step 1: Data Collection
Utilize AI-driven tools to gather real-time data on network performance metrics, including latency, bandwidth, and packet loss.
- Example Tools:
- NetScout
- SolarWinds Network Performance Monitor
Step 2: Data Analysis
Implement machine learning algorithms to analyze collected data for patterns and anomalies that may indicate potential QoS issues.
- Example Tools:
- IBM Watson for Network Analytics
- Cisco AI Network Analytics
Step 3: Real-Time Monitoring
Establish continuous monitoring systems that leverage AI to provide real-time insights into network performance and QoS.
- Example Tools:
- Dynatrace
- ThousandEyes
Step 4: Automated Response
Deploy AI-driven automation to respond to identified QoS issues by adjusting network parameters or rerouting traffic as needed.
- Example Tools:
- Juniper Networks Mist AI
- Arista Networks CloudVision
Step 5: Reporting and Feedback
Generate reports on QoS performance and AI effectiveness, providing insights for future improvements and adjustments in AI models.
- Example Tools:
- Tableau for Data Visualization
- Power BI
4. Continuous Improvement
Regularly review the workflow and AI tools used, incorporating feedback from stakeholders to enhance the overall QoS management process.
Keyword: real time quality of service management