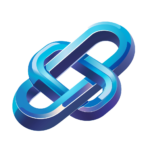
Optimize Algorithmic Trading Strategies with AI Integration
AI-driven workflow for optimizing algorithmic trading strategies includes defining objectives data acquisition model development and continuous improvement
Category: AI News Tools
Industry: Finance and Banking
Algorithmic Trading Strategy Optimization
1. Define Objectives
1.1 Establish Trading Goals
Identify specific financial targets, such as return on investment (ROI), risk tolerance, and market segments.
1.2 Determine Key Performance Indicators (KPIs)
Set measurable KPIs, including Sharpe ratio, maximum drawdown, and win/loss ratio.
2. Data Acquisition
2.1 Collect Historical Data
Utilize financial databases such as Bloomberg or Reuters for historical price data and trading volumes.
2.2 Integrate AI News Tools
Implement AI-driven news aggregation tools like AlphaSense or Bloomberg Terminal to gather relevant market sentiment and news data.
3. Data Preprocessing
3.1 Clean and Normalize Data
Use data cleaning tools such as Pandas in Python to remove inconsistencies and prepare datasets for analysis.
3.2 Feature Engineering
Generate predictive features using AI tools like DataRobot to enhance the dataset with market indicators and sentiment scores.
4. Model Development
4.1 Select Algorithm
Choose appropriate machine learning algorithms, such as Random Forest, Neural Networks, or Support Vector Machines.
4.2 Train the Model
Utilize platforms like TensorFlow or PyTorch to train the model using historical and real-time data inputs.
5. Backtesting
5.1 Implement Backtesting Framework
Employ backtesting tools such as QuantConnect or Backtrader to simulate trading strategies against historical data.
5.2 Analyze Results
Evaluate the performance of the trading strategy based on KPIs and refine the model as necessary.
6. Optimization
6.1 Parameter Tuning
Utilize optimization techniques such as Grid Search or Bayesian Optimization to fine-tune model parameters.
6.2 Ensemble Methods
Combine multiple models using ensemble methods to improve predictive accuracy and reduce overfitting.
7. Deployment
7.1 Implement Trading Strategy
Deploy the optimized trading algorithm in a live trading environment using platforms such as MetaTrader or Interactive Brokers.
7.2 Monitor Performance
Continuously track the strategy’s performance in real-time and adjust as necessary using AI-driven analytics tools like Trade Ideas.
8. Continuous Improvement
8.1 Review and Refine
Regularly review trading outcomes and market conditions to refine strategies and incorporate new data insights.
8.2 Update AI Models
Periodically retrain AI models with fresh data to adapt to changing market dynamics and improve predictive capabilities.
Keyword: algorithmic trading strategy optimization