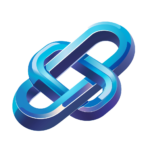
AI Integrated Workflow for Drug Discovery and Development Process
AI-driven drug discovery enhances efficiency through data collection compound screening preclinical trials clinical trials post-marketing surveillance and regulatory compliance
Category: AI News Tools
Industry: Healthcare
AI-Enhanced Drug Discovery and Development Process
1. Preliminary Research and Data Collection
1.1 Identify Disease Targets
Utilize AI algorithms to analyze existing medical literature and genomic databases to identify potential disease targets.
1.2 Data Aggregation
Employ tools like IBM Watson for Drug Discovery to gather and integrate data from various sources, including clinical trials, patient records, and scientific publications.
2. Compound Screening
2.1 Virtual Screening
Implement AI-driven platforms such as DeepChem or Atomwise for virtual screening of compound libraries to predict interactions with biological targets.
2.2 Predictive Modeling
Utilize machine learning models to predict the efficacy and safety of compounds, leveraging tools like Schrödinger or Accelrys.
3. Preclinical Development
3.1 In Silico Trials
Conduct in silico simulations using AI tools to model drug behavior and optimize dosage regimens before moving to animal studies.
3.2 Toxicology Prediction
Use AI-driven toxicology prediction tools like Tox21 to assess potential adverse effects of drug candidates.
4. Clinical Trials
4.1 Patient Recruitment
Leverage AI algorithms for patient stratification and recruitment, utilizing platforms such as TrialX to match patients with appropriate trials.
4.2 Data Monitoring and Analysis
Implement real-time data monitoring systems powered by AI, such as Medidata, to analyze trial data and ensure compliance with regulatory standards.
5. Post-Marketing Surveillance
5.1 Adverse Event Reporting
Utilize AI tools to analyze social media and electronic health records for real-time monitoring of adverse events post-launch, employing platforms like IBM Watson Health.
5.2 Continuous Improvement
Implement machine learning for ongoing analysis of drug performance and patient outcomes to inform future research and development cycles.
6. Regulatory Compliance
6.1 Documentation Automation
Use AI-driven solutions for automating the preparation of regulatory documents, ensuring compliance with guidelines set by entities like the FDA.
6.2 Predictive Analytics for Approval
Leverage predictive analytics to assess the likelihood of regulatory approval based on historical data and current submission trends.
Keyword: AI driven drug discovery process