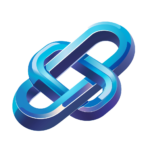
AI Driven Predictive Maintenance Workflow for Bank Properties
AI-driven predictive maintenance for bank-owned properties enhances property management through data collection analysis scheduling and performance monitoring
Category: AI Real Estate Tools
Industry: Banks and Financial Institutions
Predictive Maintenance for Bank-Owned Properties
1. Data Collection
1.1 Property Data Acquisition
Gather comprehensive data on bank-owned properties, including age, location, condition, and maintenance history.
1.2 Sensor Integration
Install IoT sensors in properties to monitor real-time conditions such as temperature, humidity, and structural integrity.
2. Data Analysis
2.1 AI-Driven Analytics Tools
Utilize AI-driven analytics platforms like IBM Watson or Microsoft Azure Machine Learning to process and analyze collected data.
2.2 Predictive Modeling
Develop predictive models using machine learning algorithms to forecast maintenance needs based on historical patterns and real-time data.
3. Maintenance Scheduling
3.1 Automated Alerts
Implement automated alert systems to notify property managers of potential maintenance issues before they escalate.
3.2 Resource Allocation
Use AI tools such as PlanGrid or UpKeep to optimize resource allocation for maintenance tasks based on predictive insights.
4. Execution of Maintenance
4.1 Vendor Management
Leverage AI platforms like ServiceTitan for efficient vendor management and scheduling of maintenance services.
4.2 Quality Assurance
Employ AI-driven inspection tools to ensure the quality of maintenance work performed, utilizing platforms like OpenSpace for visual documentation.
5. Performance Monitoring
5.1 Continuous Data Tracking
Maintain ongoing monitoring through IoT sensors to evaluate the effectiveness of maintenance efforts and adjust strategies as necessary.
5.2 Reporting and Insights
Generate reports using AI analytics tools to provide insights into maintenance performance, costs, and property conditions for strategic decision-making.
6. Feedback Loop
6.1 Stakeholder Input
Collect feedback from property managers and maintenance teams to refine predictive models and improve the overall maintenance process.
6.2 Model Refinement
Continuously update predictive models based on new data and insights to enhance accuracy and efficiency in maintenance predictions.
Keyword: Predictive maintenance for properties