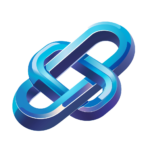
AI Driven Predictive Revenue Modeling for New Locations
AI-driven predictive revenue modeling for new locations enhances decision making through data collection analysis and continuous improvement for optimal performance
Category: AI Real Estate Tools
Industry: Retail Chains (for location selection)
Predictive Revenue Modeling for New Locations
1. Data Collection
1.1 Identify Relevant Data Sources
- Demographic Data: Age, income, population density.
- Market Trends: Local economic indicators, consumer spending habits.
- Competitor Analysis: Locations of competitors, their performance metrics.
- Geospatial Data: Accessibility, foot traffic patterns, zoning regulations.
1.2 Gather Historical Sales Data
- Collect sales data from existing locations.
- Utilize CRM systems to extract customer behavior data.
2. Data Processing
2.1 Clean and Normalize Data
- Use AI tools like DataRobot or Alteryx to clean data sets.
- Normalize data to ensure consistency across different sources.
2.2 Enrich Data with AI
- Implement AI-driven tools like IBM Watson to identify patterns and correlations.
- Utilize machine learning algorithms to predict future trends based on historical data.
3. Revenue Modeling
3.1 Develop Predictive Models
- Use AI platforms such as Google Cloud AI or Microsoft Azure Machine Learning to create predictive models.
- Incorporate factors like location characteristics, market trends, and competitor performance into the models.
3.2 Validate Models
- Test models against historical performance data to assess accuracy.
- Adjust models based on feedback and performance metrics.
4. Location Analysis
4.1 Evaluate Potential Locations
- Utilize AI-driven location analytics tools like Esri ArcGIS or SiteZeus to assess potential sites.
- Analyze foot traffic patterns, accessibility, and local competition.
4.2 Conduct Scenario Analysis
- Run simulations to forecast revenue under various scenarios using AI tools.
- Consider different market conditions, consumer behaviors, and competitor actions.
5. Decision Making
5.1 Present Findings
- Compile insights and predictive models into a comprehensive report.
- Utilize visualization tools such as Tableau or Power BI to present data effectively.
5.2 Make Informed Decisions
- Conduct stakeholder meetings to discuss findings and recommendations.
- Utilize AI-driven decision support systems to guide final location selection.
6. Implementation and Monitoring
6.1 Execute Location Strategy
- Initiate site acquisition and development based on selected locations.
- Coordinate with marketing and operations teams for launch planning.
6.2 Monitor Performance
- Utilize AI analytics tools to continuously monitor sales and customer engagement post-launch.
- Adjust strategies based on real-time data and predictive insights.
7. Continuous Improvement
7.1 Gather Feedback
- Collect feedback from customers and employees regarding new locations.
- Utilize AI sentiment analysis tools to gauge customer satisfaction.
7.2 Refine Predictive Models
- Continuously update predictive models with new data to improve accuracy.
- Incorporate lessons learned from each location launch into future models.
Keyword: Predictive revenue modeling locations