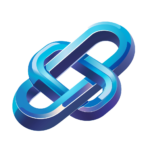
AI Driven Renewable Energy Integration and Forecasting Workflow
AI-driven workflow for renewable energy integration includes data collection analysis forecasting and system optimization for improved energy management and stakeholder engagement
Category: AI Relationship Tools
Industry: Energy and Utilities
Renewable Energy Integration and Forecasting
1. Data Collection
1.1 Identify Data Sources
Gather data from various sources including:
- Smart meters
- Weather forecasts
- Grid performance metrics
- Energy consumption patterns
1.2 Implement Data Acquisition Tools
Utilize AI-driven data collection tools such as:
- IBM Watson IoT: For real-time data ingestion from IoT devices.
- Azure IoT Hub: To connect, monitor, and manage IoT assets.
2. Data Processing and Analysis
2.1 Data Cleaning and Preparation
Ensure data accuracy by implementing:
- Data validation techniques
- Outlier detection algorithms
2.2 AI-Driven Analytics
Employ AI tools for data analysis, such as:
- Google Cloud AI: For predictive analytics and machine learning models.
- TensorFlow: To build custom forecasting models for energy demand.
3. Forecasting Energy Production and Demand
3.1 Develop Forecasting Models
Create models that predict renewable energy output and consumer demand using:
- Time series analysis
- Regression analysis
3.2 Implement AI Forecasting Tools
Utilize AI-powered forecasting tools such as:
- EnergyHub: For smart home energy management and forecasting.
- Uplight: To provide insights on customer energy usage patterns.
4. Integration with Energy Systems
4.1 Develop Integration Protocols
Establish protocols for integrating forecasting models with existing energy management systems.
4.2 Use AI for System Optimization
Implement AI solutions to optimize energy distribution, such as:
- AutoGrid: For real-time energy management and optimization.
- Siemens Spectrum Power: To enhance grid operations and reliability.
5. Monitoring and Continuous Improvement
5.1 Establish Monitoring Framework
Set up a framework for ongoing monitoring of energy production and consumption.
5.2 Utilize AI for Performance Analysis
Apply AI tools for continuous improvement, including:
- Tableau: For data visualization and performance tracking.
- Qlik Sense: To analyze trends and identify areas for optimization.
6. Reporting and Stakeholder Engagement
6.1 Generate Reports
Create comprehensive reports detailing energy forecasts and system performance.
6.2 Engage Stakeholders
Utilize AI-driven communication tools to engage stakeholders effectively, such as:
- Slack: For real-time collaboration and updates.
- Microsoft Teams: For meetings and presentations on energy forecasts.
Keyword: Renewable energy forecasting tools