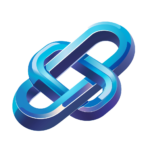
AI Driven Supply Chain Demand Forecasting Workflow Guide
AI-driven supply chain demand forecasting enhances accuracy through data collection preparation modeling and continuous improvement for optimal inventory management
Category: AI Relationship Tools
Industry: Manufacturing
Supply Chain Demand Forecasting
1. Data Collection
1.1 Identify Data Sources
Gather historical sales data, market trends, and customer feedback from various sources such as ERP systems, CRM platforms, and market research reports.
1.2 Integrate AI Tools
Utilize AI-driven data integration tools like Integromat or Zapier to streamline data collection from multiple sources.
2. Data Preparation
2.1 Data Cleaning
Employ AI algorithms to identify and rectify anomalies in the data, ensuring accuracy and consistency.
2.2 Data Enrichment
Use AI tools such as Tableau or Power BI to enhance data sets with additional insights, such as demographic information or economic indicators.
3. Demand Forecasting
3.1 Model Selection
Select appropriate AI models for demand forecasting, such as time series analysis or machine learning algorithms. Tools like Amazon Forecast or Google Cloud AI can be utilized for this purpose.
3.2 Model Training
Train the chosen models using historical data to predict future demand. This can be facilitated by platforms like TensorFlow or Azure Machine Learning.
4. Forecast Validation
4.1 Performance Evaluation
Evaluate the accuracy of the forecasts using metrics such as Mean Absolute Percentage Error (MAPE) and Root Mean Square Error (RMSE).
4.2 Continuous Improvement
Implement feedback loops using AI-driven analytics tools like Qlik or IBM Watson Analytics to continuously refine forecasting models based on real-time data.
5. Implementation of Forecasts
5.1 Inventory Management
Utilize AI-powered inventory management systems, such as NetSuite or Fishbowl, to align stock levels with demand forecasts.
5.2 Supplier Collaboration
Engage with suppliers using AI-driven relationship management tools like Salesforce or Oracle SCM Cloud to ensure timely procurement aligned with forecasted demand.
6. Monitoring and Reporting
6.1 Real-Time Monitoring
Implement real-time dashboards using tools like Microsoft Power BI or Domo to monitor demand forecasts against actual sales.
6.2 Reporting Insights
Generate comprehensive reports for stakeholders using AI analytics tools to provide insights and recommendations based on demand forecasting outcomes.
7. Feedback Loop
7.1 Collect Feedback
Gather feedback from sales and operations teams to evaluate the effectiveness of the demand forecasting process.
7.2 Iterate and Improve
Continuously refine the forecasting process by incorporating feedback and leveraging AI tools for enhanced predictive capabilities.
Keyword: AI driven supply chain forecasting