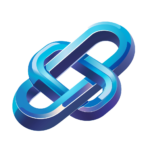
AI Driven Predictive Demand Forecasting and Replenishment Cycle
AI-driven predictive demand forecasting enhances inventory management through data collection processing modeling and continuous improvement for accurate replenishment cycles
Category: AI Research Tools
Industry: Retail and E-commerce
Predictive Demand Forecasting and Replenishment Cycle
1. Data Collection
1.1. Historical Sales Data
Gather historical sales data from various sources, including point-of-sale systems and e-commerce platforms.
1.2. Market Trends Analysis
Utilize tools such as Google Trends and social media analytics to identify current market trends and consumer preferences.
1.3. External Factors
Incorporate data on seasonality, promotions, and economic indicators to enhance forecasting accuracy.
2. Data Processing
2.1. Data Cleaning
Use AI-driven data cleaning tools like Trifacta to ensure data accuracy and consistency.
2.2. Data Integration
Combine data from various sources into a centralized database using ETL (Extract, Transform, Load) processes.
3. Predictive Modeling
3.1. AI Algorithm Selection
Choose appropriate AI algorithms such as ARIMA, Prophet, or machine learning models like Random Forest and Neural Networks for demand forecasting.
3.2. Model Training
Train the selected models using historical data to predict future demand patterns.
3.3. Model Evaluation
Evaluate model performance using metrics such as Mean Absolute Error (MAE) and Root Mean Square Error (RMSE) to ensure accuracy.
4. Demand Forecasting
4.1. Forecast Generation
Generate demand forecasts for different product categories using the trained models.
4.2. Visualization Tools
Utilize visualization tools like Tableau or Power BI to present forecast data in an easily digestible format for stakeholders.
5. Inventory Replenishment Planning
5.1. Replenishment Strategies
Develop replenishment strategies based on forecasted demand, including Just-In-Time (JIT) and Economic Order Quantity (EOQ) methods.
5.2. Automated Replenishment Tools
Implement AI-driven inventory management systems such as TradeGecko or Netstock to automate replenishment processes.
6. Monitoring and Adjustment
6.1. Performance Tracking
Continuously monitor inventory levels and sales performance against forecasts using dashboards.
6.2. Feedback Loop
Establish a feedback loop to refine forecasting models based on discrepancies between predicted and actual sales.
7. Reporting and Analysis
7.1. Executive Reporting
Generate regular reports for management to review forecast accuracy and inventory performance.
7.2. Continuous Improvement
Utilize insights gained from analysis to improve future forecasting accuracy and inventory management practices.
Keyword: AI driven demand forecasting