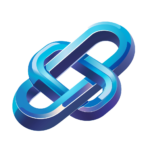
Automated AML Monitoring with AI Integration for Enhanced Compliance
Automated AML monitoring leverages AI for data collection risk assessment alert generation investigation reporting and continuous improvement in compliance efforts
Category: AI Security Tools
Industry: Financial Services
Automated Anti-Money Laundering (AML) Monitoring
1. Data Collection
1.1 Source Identification
Identify and integrate various data sources, such as:
- Customer transaction data
- Account opening information
- Public records and watchlists
1.2 Data Integration
Utilize AI-driven data integration tools to consolidate data from multiple sources into a centralized database.
- Example Tool: Apache Nifi
- Example Tool: Talend
2. Risk Assessment
2.1 Customer Risk Profiling
Implement AI algorithms to analyze customer profiles and assign risk ratings based on various factors, including:
- Transaction patterns
- Geographic location
- Type of business activity
2.2 Transaction Monitoring
Deploy machine learning models to monitor transactions in real-time, identifying anomalies and potential red flags.
- Example Tool: Actimize
- Example Tool: SAS Anti-Money Laundering
3. Alert Generation
3.1 Anomaly Detection
Utilize AI-driven systems to generate alerts for suspicious activities based on predefined thresholds and learned behavior.
3.2 Alert Prioritization
Implement AI to prioritize alerts based on risk levels and potential impact, ensuring compliance teams focus on high-risk cases first.
4. Investigation
4.1 Automated Case Management
Use AI tools to automate the case management process, tracking investigations and documenting findings efficiently.
- Example Tool: Amlify
- Example Tool: ComplyAdvantage
4.2 Human Oversight
Ensure that compliance officers review and validate AI-generated alerts and investigations to maintain regulatory standards.
5. Reporting
5.1 Regulatory Reporting
Automate the generation of reports required for regulatory compliance using AI-driven reporting tools.
- Example Tool: Oracle Financial Services Analytical Applications
- Example Tool: FICO TONBELLER
5.2 Performance Analytics
Leverage AI analytics to assess the effectiveness of the AML monitoring process and identify areas for improvement.
6. Continuous Improvement
6.1 Model Training
Regularly update machine learning models with new data to enhance detection capabilities and adapt to evolving money laundering techniques.
6.2 Feedback Loop
Create a feedback mechanism where compliance teams can provide insights on false positives and false negatives to refine AI algorithms.
Keyword: automated anti-money laundering monitoring