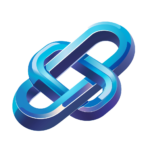
AI Integration in Adaptive Smart Grid Management Workflow
Discover AI-driven smart grid management with real-time data collection analysis optimization and continuous improvement for efficient sustainable energy solutions
Category: AI Self Improvement Tools
Industry: Environmental and Climate Tech
Adaptive Smart Grid Management
1. Assessment and Data Collection
1.1 Identify Key Performance Indicators (KPIs)
Define the metrics that will measure the efficiency and sustainability of the smart grid.
1.2 Deploy IoT Sensors
Implement Internet of Things (IoT) sensors across the grid to collect real-time data on energy consumption, generation, and environmental conditions.
1.3 Data Aggregation
Utilize AI-driven data aggregation tools to compile data from various sources, ensuring a comprehensive dataset.
2. Data Analysis and Insights Generation
2.1 AI-Driven Predictive Analytics
Employ predictive analytics tools such as IBM Watson or Google Cloud AI to forecast energy demand and identify potential outages before they occur.
2.2 Machine Learning Algorithms
Implement machine learning algorithms to analyze historical data and optimize energy distribution based on usage patterns.
2.3 Environmental Impact Assessment
Utilize AI tools like EnerNOC to evaluate the environmental impact of energy production and consumption, helping to identify areas for improvement.
3. Optimization and Decision Making
3.1 Real-Time Monitoring Systems
Integrate AI-powered real-time monitoring systems to adjust energy distribution dynamically based on current demand and supply conditions.
3.2 Automated Demand Response
Implement automated demand response systems that utilize AI to manage energy loads during peak times, ensuring grid stability.
3.3 Resource Allocation
Use AI tools to optimize resource allocation, ensuring that renewable energy sources are utilized effectively, reducing reliance on fossil fuels.
4. Implementation of AI Self-Improvement Tools
4.1 Continuous Learning Systems
Deploy AI systems that continuously learn from operational data, improving their algorithms over time to enhance grid management.
4.2 Feedback Loops
Establish feedback loops where AI systems can receive input from human operators, refining decision-making processes based on real-world outcomes.
4.3 Collaboration with AI Platforms
Partner with AI platforms such as Microsoft Azure AI or Amazon SageMaker to leverage advanced machine learning capabilities for ongoing system improvements.
5. Reporting and Stakeholder Engagement
5.1 Generate Reports
Create comprehensive reports detailing energy usage, environmental impact, and system performance using AI-driven reporting tools.
5.2 Stakeholder Communication
Utilize AI chatbots and communication tools to engage stakeholders and provide them with real-time updates and insights on grid performance.
5.3 Policy Recommendations
Analyze data to formulate policy recommendations that support sustainable energy practices and enhance regulatory compliance.
6. Continuous Improvement
6.1 Performance Review
Conduct regular performance reviews of the smart grid management system, identifying areas for further enhancement.
6.2 Update AI Algorithms
Regularly update AI algorithms based on new data and findings to ensure optimal performance and adaptability to changing conditions.
6.3 Stakeholder Feedback Integration
Incorporate feedback from stakeholders to refine processes and improve overall grid management strategies.
Keyword: adaptive smart grid management