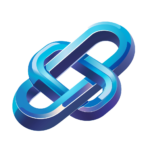
AI Driven Smart Grid Performance Analysis Workflow Guide
AI-driven smart grid performance analysis enhances data collection preprocessing analysis monitoring reporting and continuous improvement for optimal grid efficiency
Category: AI Summarizer Tools
Industry: Energy and Utilities
Smart Grid Performance Analysis
1. Data Collection
1.1 Identify Data Sources
Gather data from various sources including smart meters, sensors, and grid management systems.
1.2 Data Acquisition
Utilize IoT devices for real-time data collection. Examples include:
- Smart Meters
- Phasor Measurement Units (PMUs)
2. Data Preprocessing
2.1 Data Cleaning
Remove inaccuracies and fill in gaps in the dataset using AI algorithms.
2.2 Data Normalization
Standardize data formats to ensure consistency across datasets.
3. Data Analysis
3.1 Descriptive Analysis
Utilize AI-driven analytics tools to summarize historical performance data.
- Example Tool: Tableau
3.2 Predictive Analysis
Implement machine learning models to forecast future grid performance and potential outages.
- Example Tool: IBM Watson Studio
4. Performance Monitoring
4.1 Real-time Monitoring
Deploy AI-powered dashboards to visualize performance metrics in real-time.
- Example Tool: Microsoft Power BI
4.2 Anomaly Detection
Use AI algorithms to detect anomalies in grid performance, alerting operators to potential issues.
- Example Tool: Google Cloud AI
5. Reporting
5.1 Automated Reporting
Generate automated reports summarizing performance analysis and insights using AI summarization tools.
- Example Tool: OpenAI’s GPT-3
5.2 Stakeholder Communication
Distribute reports to stakeholders, including actionable insights and recommendations for improvements.
6. Continuous Improvement
6.1 Feedback Loop
Integrate feedback from stakeholders to refine data collection and analysis processes.
6.2 Model Refinement
Regularly update AI models based on new data and performance outcomes to enhance accuracy.
Keyword: smart grid performance analysis