Namecheap Name Generator
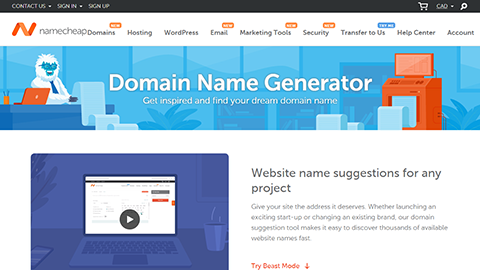
The Namecheap Name Generator is an AI-powered domain name generator designed to assist entrepreneurs, web developers, and small business owners in finding available domain names based on their chosen keywords. This user-friendly website naming tool employs advanced algorithms to provide a diverse array of domain suggestions across multiple extensions, making it easier to discover alternatives if a preferred .com domain is unavailable. With instant domain availability and pricing information, users can quickly assess their options, while the integration with Namecheap’s domain registration services streamlines the purchasing process. Although some generated names may lack creativity compared to specialized naming tools, the Namecheap domain finder remains a practical solution for those seeking affordable domains and exploring various domain extensions. Additional features, such as the Beast Mode for expanded suggestions, enhance the overall experience, although the tool may prioritize availability over brand name creation and offer limited customization options.