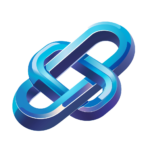
AI Driven Medical Research Data Extraction Workflow Guide
AI-driven medical research data extraction workflow enhances efficiency by automating data collection preprocessing analysis and implementation of findings
Category: AI Transcription Tools
Industry: Healthcare
Medical Research Data Extraction Workflow
1. Define Objectives and Scope
1.1 Identify Research Goals
Determine the specific medical research questions that need to be addressed.
1.2 Establish Data Requirements
Outline the types of data required for analysis, including patient records, clinical trial results, and published studies.
2. Data Collection
2.1 Source Identification
Identify reliable sources of medical data, such as electronic health records (EHRs), medical journals, and clinical databases.
2.2 Data Acquisition
Utilize AI-driven tools to automate the collection of data. Examples include:
- Google Cloud Healthcare API – Facilitates the integration of healthcare data from various sources.
- IBM Watson Health – Provides access to a vast array of healthcare data for analysis.
3. Data Preprocessing
3.1 Data Cleaning
Implement AI algorithms to identify and rectify inaccuracies or inconsistencies in the dataset.
3.2 Data Structuring
Transform unstructured data into structured formats suitable for analysis using tools like:
- Amazon Comprehend Medical – Extracts relevant medical information from unstructured text.
- Microsoft Azure Text Analytics – Analyzes and organizes healthcare data for better accessibility.
4. Data Extraction
4.1 Utilize AI Transcription Tools
Employ AI transcription tools to convert audio recordings of research interviews or discussions into text. Recommended tools include:
- Otter.ai – Provides real-time transcription and collaboration features.
- Rev.ai – Offers accurate transcription services with a focus on medical terminology.
4.2 Extract Relevant Information
Use natural language processing (NLP) techniques to extract key data points from the transcribed text.
5. Data Analysis
5.1 Statistical Analysis
Employ statistical software and AI algorithms to analyze the extracted data for trends and insights.
5.2 Visualization
Utilize data visualization tools to present findings effectively. Examples include:
- Tableau – Enables interactive data visualization and dashboard creation.
- Power BI – Provides comprehensive data analytics and visualization capabilities.
6. Reporting and Review
6.1 Compile Findings
Summarize the results of the analysis in a structured report.
6.2 Peer Review
Engage stakeholders in a review process to validate findings and conclusions.
7. Implementation of Findings
7.1 Develop Action Plans
Create actionable strategies based on the research findings.
7.2 Monitor Outcomes
Establish metrics to evaluate the impact of implemented strategies over time.
8. Continuous Improvement
8.1 Feedback Loop
Incorporate feedback from stakeholders to refine the data extraction process.
8.2 Update Tools and Techniques
Regularly assess and update AI tools and methodologies to enhance efficiency and accuracy.
Keyword: medical research data extraction