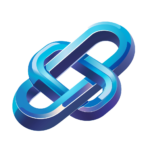
AI Integration in Drug Discovery and Development Workflow
Discover how AI-driven workflows enhance drug discovery and development from research to post-market surveillance optimizing efficiency and safety in pharmaceuticals
Category: AI Website Tools
Industry: Healthcare
AI-Driven Drug Discovery and Development Pipeline
1. Initial Research and Data Collection
1.1 Literature Review
Utilize AI-powered tools such as IBM Watson Discovery to analyze vast amounts of scientific literature and identify relevant studies.
1.2 Data Gathering
Employ PubMed API and ClinicalTrials.gov to collect data on existing drugs, clinical trials, and patient outcomes.
2. Target Identification
2.1 Biomarker Discovery
Implement machine learning algorithms through platforms like DeepMind’s AlphaFold to predict protein structures and identify potential drug targets.
2.2 Genetic Profiling
Use Tempus for genomic data analysis to discover genetic mutations associated with diseases.
3. Compound Screening
3.1 Virtual Screening
Leverage AI tools like Schrödinger and OpenEye Scientific Software to simulate interactions between drug candidates and targets.
3.2 High-Throughput Screening
Incorporate robotics and AI algorithms to automate the testing of thousands of compounds for biological activity.
4. Lead Optimization
4.1 Structure-Activity Relationship (SAR) Modeling
Utilize AI-driven predictive modeling tools such as ChemAxon to optimize lead compounds based on their biological activity.
4.2 ADMET Prediction
Employ AI tools like QikProp to predict Absorption, Distribution, Metabolism, Excretion, and Toxicity (ADMET) profiles of compounds.
5. Preclinical Development
5.1 In Vitro and In Vivo Testing
Use AI analytics platforms like BioSymetrics to analyze biological data from preclinical studies and optimize experimental designs.
5.2 Data Integration
Implement LabArchives for data management and integration to streamline collaboration among research teams.
6. Clinical Trials
6.1 Patient Recruitment
Utilize AI-driven platforms like Antidote to match patients with clinical trials based on their genetic profiles and health records.
6.2 Trial Monitoring
Incorporate AI tools such as Medidata for real-time data analytics and monitoring of clinical trial progress.
7. Regulatory Submission
7.1 Documentation Preparation
Use AI-assisted document management systems like Veeva Vault to streamline the preparation of regulatory submissions.
7.2 Compliance Monitoring
Implement AI tools to ensure compliance with regulatory standards and automate reporting processes.
8. Post-Market Surveillance
8.1 Pharmacovigilance
Leverage AI platforms like Oracle’s Argus Safety to monitor drug safety and adverse effects in the market.
8.2 Continuous Improvement
Use machine learning algorithms to analyze post-market data and inform future drug development strategies.
Keyword: AI driven drug discovery pipeline