Predictive Analytics and AI Agents for Demand Forecasting
Topic: AI Agents
Industry: Logistics and Supply Chain
Discover how predictive analytics and AI agents enhance demand forecasting in supply chain management to improve efficiency and reduce costs in volatile markets.
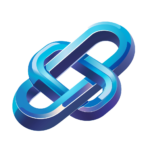
Predictive Analytics and AI Agents: Forecasting Demand in Volatile Markets
Understanding the Role of Predictive Analytics in Supply Chain Management
In today’s fast-paced business environment, the ability to accurately forecast demand is essential for effective supply chain management. Predictive analytics, powered by artificial intelligence (AI), offers organizations the tools to anticipate market fluctuations, optimize inventory levels, and enhance overall operational efficiency. By leveraging historical data and advanced algorithms, businesses can gain insights that drive informed decision-making.The Importance of AI Agents in Logistics
AI agents have emerged as vital components in the logistics and supply chain sectors. These intelligent systems can analyze vast amounts of data in real-time, enabling organizations to respond swiftly to changes in demand. By automating routine tasks and providing actionable insights, AI agents help businesses streamline operations and reduce costs.Key Benefits of Implementing AI in Demand Forecasting
1. Enhanced Accuracy: AI algorithms can process complex datasets, leading to more precise demand forecasts compared to traditional methods. 2. Real-Time Insights: AI agents provide real-time analytics, allowing businesses to adjust supply chain strategies on the fly. 3. Cost Efficiency: By optimizing inventory levels and reducing waste, AI-driven solutions can significantly lower operational costs. 4. Improved Customer Satisfaction: Accurate demand forecasting ensures that products are available when customers need them, enhancing overall satisfaction and loyalty.Tools and Technologies for Effective Demand Forecasting
To harness the power of predictive analytics and AI agents, organizations can implement a variety of tools and technologies. Here are some notable examples:1. IBM Watson Supply Chain
IBM Watson Supply Chain utilizes AI to provide insights into supply chain operations. Its predictive analytics capabilities allow businesses to forecast demand, manage risks, and optimize inventory levels. The platform integrates seamlessly with existing systems, offering a comprehensive view of supply chain dynamics.2. Microsoft Azure Machine Learning
Microsoft Azure Machine Learning is a cloud-based service that empowers businesses to build, train, and deploy machine learning models. By utilizing historical sales data, organizations can create custom demand forecasting models tailored to their specific needs. The platform’s scalability makes it suitable for businesses of all sizes.3. SAP Integrated Business Planning (IBP)
SAP IBP provides a unified platform for demand planning, supply planning, and inventory optimization. Its advanced analytics capabilities leverage machine learning to enhance forecasting accuracy. Additionally, SAP IBP offers collaboration tools that enable teams to work together effectively, ensuring alignment across the supply chain.4. Oracle Demand Management Cloud
Oracle Demand Management Cloud leverages AI to improve demand forecasting and planning processes. With its ability to analyze market trends and customer behavior, the platform helps businesses make data-driven decisions. Oracle’s solution also integrates with other Oracle Cloud applications, providing a holistic approach to supply chain management.Implementing AI Agents: Best Practices
To successfully implement AI agents for demand forecasting, organizations should consider the following best practices:1. Data Quality and Integration
Ensure that the data used for predictive analytics is accurate, complete, and integrated from various sources. High-quality data is the foundation for reliable forecasts.2. Continuous Learning
AI systems should be designed to learn continuously from new data. Regularly updating models will enhance their accuracy and adaptability to changing market conditions.3. Collaboration Across Teams
Foster collaboration between IT, operations, and business teams to ensure that insights from AI agents are effectively utilized in decision-making processes.4. Monitor and Evaluate Performance
Establish key performance indicators (KPIs) to monitor the effectiveness of AI-driven demand forecasting. Regular evaluation will help identify areas for improvement and optimize the use of AI agents.Conclusion
In conclusion, predictive analytics and AI agents are transforming the landscape of logistics and supply chain management. By harnessing these technologies, organizations can improve demand forecasting, enhance operational efficiency, and ultimately drive business success in volatile markets. As the industry continues to evolve, embracing AI-driven solutions will be crucial for staying competitive and meeting the ever-changing demands of consumers.Keyword: AI demand forecasting solutions