AI Agents in Predictive Maintenance 2.0 Preventing Downtime
Topic: AI Agents
Industry: Manufacturing
Discover how AI agents in Predictive Maintenance 2.0 enhance efficiency reduce downtime and optimize operations for manufacturers in the digital age
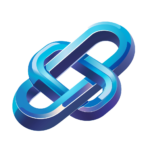
Predictive Maintenance 2.0: How AI Agents are Preventing Downtime
Understanding Predictive Maintenance
Predictive maintenance represents a transformative shift in the way manufacturing industries approach equipment upkeep. By leveraging data analytics and machine learning, organizations can anticipate equipment failures before they occur, thereby minimizing unplanned downtime and optimizing operational efficiency. With the advent of AI agents, predictive maintenance has evolved into a more proactive and intelligent process—termed Predictive Maintenance 2.0.
The Role of AI Agents in Predictive Maintenance
AI agents are software applications that utilize artificial intelligence to analyze data and make decisions. In the context of predictive maintenance, these agents can monitor equipment performance, identify patterns, and predict potential failures. By integrating AI agents into maintenance protocols, manufacturers can significantly reduce costs associated with downtime and repairs.
Key Benefits of Implementing AI Agents
- Enhanced Predictive Accuracy: AI algorithms can analyze vast datasets far more efficiently than human operators, resulting in more accurate predictions of equipment failures.
- Real-Time Monitoring: AI agents continuously monitor machine performance, allowing for immediate detection of anomalies that may indicate impending issues.
- Resource Optimization: By predicting maintenance needs, organizations can schedule interventions during planned downtimes, ensuring that resources are utilized effectively.
Implementation of AI in Predictive Maintenance
Implementing AI-driven solutions for predictive maintenance involves several key steps:
1. Data Collection
To leverage AI agents effectively, manufacturers must first gather data from various sources, including sensors, IoT devices, and historical maintenance records. This data serves as the foundation for AI algorithms to analyze and learn from.
2. Data Analysis and Model Training
Once data is collected, AI models are trained to identify patterns and correlations that can predict equipment failures. Machine learning techniques, such as supervised and unsupervised learning, are commonly employed for this purpose.
3. Integration with Existing Systems
AI agents must be integrated with existing manufacturing systems and workflows. This requires collaboration between IT and operational teams to ensure seamless communication between AI tools and machinery.
4. Continuous Improvement
The deployment of AI agents is not a one-time effort. Continuous monitoring and feedback loops are essential to refine algorithms and improve predictive accuracy over time.
Examples of AI-Driven Tools for Predictive Maintenance
Several tools and platforms are leading the way in integrating AI into predictive maintenance:
IBM Maximo
IBM Maximo utilizes AI to enhance asset management and predictive maintenance capabilities. Its AI-driven analytics allow manufacturers to predict equipment failures and optimize maintenance schedules, thereby reducing downtime.
Uptake
Uptake is an AI-powered asset performance management platform that provides actionable insights based on real-time data. Its predictive maintenance solutions help organizations anticipate failures and extend asset life.
Siemens MindSphere
Siemens MindSphere is an industrial IoT platform that harnesses AI to analyze data from connected machines. By leveraging machine learning algorithms, it enables predictive maintenance and operational optimization.
Conclusion
As manufacturing industries continue to embrace digital transformation, the integration of AI agents into predictive maintenance strategies will play a crucial role in enhancing operational efficiency. By leveraging advanced analytics and real-time monitoring, organizations can minimize downtime, reduce costs, and ultimately drive greater productivity. Predictive Maintenance 2.0 is not just a trend; it is a strategic imperative for manufacturers looking to thrive in a competitive landscape.
Keyword: AI predictive maintenance solutions