Transforming Yield Forecasting with Predictive Analytics for Farmers
Topic: AI Analytics Tools
Industry: Agriculture
Discover how predictive analytics and AI are transforming yield forecasting for farmers enhancing productivity and sustainability in agriculture.
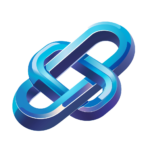
How Predictive Analytics is Transforming Yield Forecasting for Farmers
The Role of Predictive Analytics in Agriculture
In the rapidly evolving landscape of agriculture, predictive analytics stands out as a pivotal tool for enhancing yield forecasting. By leveraging data-driven insights, farmers can make informed decisions that significantly impact their productivity and profitability. With the integration of artificial intelligence (AI), these analytics tools are becoming more sophisticated, enabling farmers to anticipate crop outcomes with remarkable accuracy.
Understanding Predictive Analytics
Predictive analytics involves the use of statistical algorithms and machine learning techniques to identify the likelihood of future outcomes based on historical data. In agriculture, this means analyzing factors such as weather patterns, soil conditions, crop health, and market trends to forecast yields. The insights gained can help farmers optimize their operations, reduce waste, and maximize their returns.
Key Benefits of Predictive Analytics for Farmers
- Enhanced Decision-Making: By providing actionable insights, predictive analytics empowers farmers to make data-driven decisions regarding planting schedules, resource allocation, and crop management.
- Risk Mitigation: With the ability to foresee potential challenges, such as pest infestations or adverse weather conditions, farmers can implement preventive measures to safeguard their crops.
- Resource Optimization: Predictive models can help farmers allocate resources more efficiently, reducing costs and minimizing environmental impact.
Implementing AI in Yield Forecasting
The implementation of AI in predictive analytics is revolutionizing yield forecasting. By harnessing vast amounts of data, AI algorithms can identify patterns and correlations that may go unnoticed by human analysts. Here are several ways AI can be integrated into yield forecasting:
1. Data Collection and Processing
AI-driven tools can automate the collection and processing of data from various sources, including satellite imagery, IoT sensors, and historical yield records. This comprehensive data gathering ensures that farmers have access to the most relevant information for accurate forecasting.
2. Machine Learning Models
Machine learning algorithms can analyze historical data to develop predictive models that forecast yields based on current conditions. These models can continuously improve as they process more data, becoming increasingly accurate over time.
3. Real-Time Analytics
AI tools can provide real-time analytics, allowing farmers to monitor their crops continuously. This capability enables immediate responses to emerging issues, such as nutrient deficiencies or pest outbreaks, ultimately protecting yield potential.
Examples of AI-Driven Tools for Yield Forecasting
Several AI-driven products are currently making waves in the agricultural sector, providing farmers with powerful tools for yield forecasting:
1. Climate Corporation
Climate Corporation offers a suite of data-driven solutions that utilize predictive analytics to help farmers optimize their yields. Their Climate FieldView platform provides insights into field variability, enabling farmers to make informed decisions about planting and management practices.
2. IBM Watson Decision Platform for Agriculture
IBM’s Watson Decision Platform integrates AI, weather data, and IoT to deliver actionable insights for farmers. By analyzing data from various sources, it helps farmers anticipate yield outcomes and manage risks effectively.
3. Granular
Granular is a farm management software that leverages predictive analytics to provide farmers with insights into their operations. By analyzing historical data and current conditions, Granular helps farmers forecast yields and make data-driven decisions.
Conclusion
The integration of predictive analytics and AI in agriculture is transforming yield forecasting, enabling farmers to enhance their productivity and sustainability. By leveraging these advanced tools, farmers can make informed decisions that not only improve their yield outcomes but also contribute to a more efficient and resilient agricultural sector. As technology continues to evolve, the potential for predictive analytics in agriculture will only grow, paving the way for a new era of farming.
Keyword: predictive analytics for farmers