Key AI Analytics Platforms Driving Autonomous Vehicle Innovation
Topic: AI Analytics Tools
Industry: Automotive
Explore how AI analytics platforms like NVIDIA DRIVE Waymo and Tesla Autopilot are transforming autonomous vehicle development and shaping the future of mobility
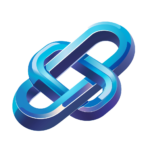
Autonomous Vehicle Development: Key AI Analytics Platforms Shaping the Future of Mobility
Understanding the Role of AI in Autonomous Vehicles
The automotive industry is undergoing a transformative shift, driven largely by advancements in artificial intelligence (AI) and machine learning technologies. As manufacturers and tech companies race to develop autonomous vehicles, AI analytics platforms have emerged as crucial tools for optimizing performance, enhancing safety, and improving user experience. These platforms leverage vast amounts of data to create actionable insights that inform decision-making throughout the development process.
AI Analytics Tools: A Closer Look
Various AI analytics tools are currently at the forefront of autonomous vehicle development. These tools not only facilitate the collection and analysis of data but also support the integration of AI algorithms into vehicle systems. Here are some notable examples:
1. NVIDIA DRIVE
NVIDIA DRIVE is a comprehensive AI platform designed specifically for autonomous vehicles. It utilizes deep learning algorithms to process data from sensors such as cameras, LiDAR, and radar. This platform enables real-time decision-making, allowing vehicles to navigate complex environments safely. Its simulation tools, like NVIDIA DRIVE Sim, further enhance the development process by allowing engineers to test and validate algorithms in virtual environments before deploying them on actual roads.
2. Waymo’s AI Software
Waymo, a leader in autonomous driving technology, employs a sophisticated AI software stack that integrates various machine learning models. This software processes data from the vehicle’s sensors to detect and classify objects, predict their behavior, and make driving decisions. Waymo’s approach emphasizes the importance of a robust training dataset, which is crucial for improving the accuracy and reliability of its self-driving systems.
3. Tesla Autopilot
Tesla’s Autopilot is another prominent example of AI analytics in action. It utilizes a combination of computer vision and neural networks to interpret data from its suite of onboard sensors. Tesla continuously collects data from its fleet, which is then analyzed to improve the Autopilot system’s performance. This data-driven approach allows for rapid updates and enhancements, ensuring that Tesla vehicles benefit from the latest advancements in AI technology.
Implementing AI Analytics in Autonomous Vehicle Development
Implementing AI analytics in the development of autonomous vehicles involves several key steps:
Data Collection
The first step is to gather extensive datasets from various sources, including vehicle sensors, traffic patterns, and environmental conditions. This data serves as the foundation for training AI models.
Model Training
Once data is collected, machine learning algorithms are employed to train models that can recognize patterns and make predictions. This phase often involves iterative testing and refinement to enhance the model’s accuracy.
Simulation and Testing
Before deploying AI models in real-world scenarios, they undergo rigorous testing in simulated environments. This allows developers to identify potential issues and ensure that the models can operate safely and effectively under various conditions.
Deployment
After thorough testing, the AI models are integrated into the vehicle’s systems. Continuous monitoring and data analysis post-deployment are essential to ensure ongoing performance improvements and safety enhancements.
The Future of Mobility
As the automotive industry continues to embrace AI analytics, the future of mobility looks promising. The integration of advanced AI tools will not only pave the way for safer and more efficient autonomous vehicles but also contribute to the development of smart transportation systems that can adapt to the needs of urban environments. Companies investing in these technologies are likely to lead the charge in redefining how we think about transportation.
Conclusion
The evolution of autonomous vehicles is heavily reliant on AI analytics platforms that provide the necessary tools for data-driven decision-making. By leveraging advanced technologies such as NVIDIA DRIVE, Waymo’s AI software, and Tesla’s Autopilot, the automotive industry is poised to revolutionize mobility. As we move forward, the collaboration between AI and automotive innovation will undoubtedly shape a safer, smarter, and more efficient future for all.
Keyword: autonomous vehicle AI analytics