Top 5 AI Predictive Maintenance Tools for Utilities Success
Topic: AI Analytics Tools
Industry: Energy and Utilities
Discover the top 5 AI-powered predictive maintenance tools for utilities that enhance efficiency reduce costs and improve service reliability in the energy sector
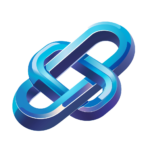
Top 5 AI-Powered Predictive Maintenance Tools for Utilities
In the rapidly evolving landscape of energy and utilities, predictive maintenance has emerged as a critical component for operational efficiency and cost reduction. By leveraging artificial intelligence (AI), utility companies can anticipate equipment failures, optimize maintenance schedules, and enhance overall service reliability. In this article, we will explore the top five AI-powered predictive maintenance tools available for utilities, highlighting their features and implementation strategies.
1. IBM Maximo Asset Health Insights
IBM Maximo Asset Health Insights utilizes AI to analyze asset health data in real-time. This tool offers predictive analytics capabilities that allow utilities to identify potential failures before they occur. By integrating IoT data and machine learning algorithms, Maximo provides actionable insights that help organizations prioritize maintenance tasks based on urgency and impact.
Implementation
To implement IBM Maximo, utilities should start by integrating their existing asset management systems with the platform. This may involve data cleansing and standardization to ensure accurate analytics. Once set up, operators can leverage the tool’s dashboard to monitor asset health and receive alerts on potential issues.
2. Siemens MindSphere
Siemens MindSphere is an open cloud-based IoT operating system that enables utilities to connect their assets and analyze data using AI algorithms. This platform provides predictive maintenance capabilities by assessing equipment performance and predicting failures based on historical data patterns.
Implementation
Utilities can implement MindSphere by connecting their devices and sensors to the platform. The data collected can be processed using Siemens’ advanced analytics tools, allowing for predictive insights. Training staff to interpret the data and take proactive measures is also essential for maximizing the platform’s benefits.
3. GE Digital’s Predix
GE Digital’s Predix platform is designed specifically for industrial IoT applications and offers robust predictive maintenance features. By utilizing machine learning and advanced analytics, Predix helps utilities monitor equipment health and predict failures, ultimately reducing downtime and maintenance costs.
Implementation
To leverage Predix, utilities should begin by deploying sensors on critical assets to gather data. The platform’s analytics capabilities can then be utilized to create predictive models that inform maintenance schedules and operational strategies. Regular training sessions for staff on data interpretation and decision-making are recommended.
4. Uptake
Uptake is an AI-driven analytics platform that focuses on predictive maintenance for various industries, including utilities. By analyzing data from multiple sources, Uptake provides insights that help organizations understand the health of their assets and predict future failures.
Implementation
Utilities can implement Uptake by integrating it with their existing data sources, such as SCADA systems and maintenance logs. The platform’s user-friendly interface allows operators to visualize data and receive recommendations for maintenance actions based on predictive analytics.
5. SAS Predictive Maintenance
SAS offers a comprehensive predictive maintenance solution that combines AI and advanced analytics to help utilities optimize their maintenance strategies. This tool allows organizations to predict equipment failures and improve asset utilization, thereby enhancing operational efficiency.
Implementation
To implement SAS Predictive Maintenance, utilities should focus on data integration from various operational sources. Utilizing SAS’s powerful analytics tools, organizations can develop predictive models tailored to their specific equipment and operational needs. Continuous monitoring and adjustment of these models will ensure ongoing accuracy and effectiveness.
Conclusion
As the energy and utilities sector continues to embrace digital transformation, AI-powered predictive maintenance tools will play a pivotal role in enhancing operational efficiency and reliability. By implementing the tools mentioned above, utilities can not only reduce maintenance costs but also improve service delivery to their customers. The future of predictive maintenance lies in the effective integration of AI analytics, enabling organizations to stay ahead of potential issues and drive sustainable growth.
Keyword: AI predictive maintenance tools for utilities