Predictive Analytics in Finance Using AI for Market Trends
Topic: AI Analytics Tools
Industry: Finance and Banking
Discover how predictive analytics powered by AI transforms finance by anticipating market trends enhancing decision-making and driving growth in financial institutions
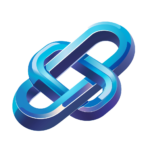
Predictive Analytics in Finance: Using AI to Anticipate Market Trends
The Evolution of Predictive Analytics in Finance
In the fast-paced world of finance and banking, the ability to anticipate market trends is invaluable. Predictive analytics, powered by artificial intelligence (AI), has emerged as a transformative force in this sector. By leveraging vast amounts of data, financial institutions can make informed decisions, mitigate risks, and identify new opportunities. This article explores how AI-driven analytics tools can be implemented in finance to enhance predictive capabilities and drive strategic outcomes.
Understanding Predictive Analytics
Predictive analytics involves using statistical algorithms and machine learning techniques to analyze historical data and predict future events. In finance, this can mean anything from forecasting stock prices to assessing credit risk. By utilizing AI, financial institutions can process and analyze data at an unprecedented scale and speed, allowing for more accurate predictions and insights.
Key Components of AI in Predictive Analytics
The integration of AI into predictive analytics encompasses several key components:
- Data Collection: Gathering historical and real-time data from various sources, including market trends, economic indicators, and social media sentiment.
- Data Processing: Utilizing AI algorithms to clean and organize data, making it suitable for analysis.
- Model Development: Creating predictive models using machine learning techniques that can identify patterns and trends.
- Continuous Learning: Implementing models that evolve over time as new data becomes available, enhancing accuracy and reliability.
Implementation of AI Analytics Tools in Finance
Implementing AI analytics tools in finance requires a strategic approach. Here are some steps financial institutions can take to effectively integrate these technologies:
1. Identify Objectives
Organizations must first define their goals for using predictive analytics. Whether it’s improving customer segmentation, enhancing risk management, or optimizing investment strategies, having clear objectives will guide the implementation process.
2. Choose the Right Tools
Selecting the appropriate AI-driven tools is crucial for success. Here are a few notable examples:
- IBM Watson Analytics: This tool offers advanced data visualization and predictive analytics capabilities, enabling finance professionals to uncover insights and trends in their data.
- Alteryx: Alteryx provides a comprehensive platform for data preparation, blending, and advanced analytics, allowing users to build predictive models without extensive coding knowledge.
- Tableau: Known for its powerful data visualization capabilities, Tableau also incorporates predictive analytics features that help finance teams visualize trends and forecast future performance.
- DataRobot: This automated machine learning platform simplifies the process of building and deploying predictive models, making it accessible for finance professionals with varying levels of expertise.
3. Train Staff and Foster a Data-Driven Culture
For AI analytics tools to be effective, staff must be trained to use these technologies effectively. Fostering a culture that values data-driven decision-making is essential for maximizing the benefits of predictive analytics.
4. Monitor and Adjust
Once implemented, it is important to continuously monitor the performance of predictive models and make adjustments as necessary. This iterative process ensures that the models remain relevant and accurate as market conditions change.
Real-World Applications of Predictive Analytics in Finance
Several financial institutions have successfully harnessed predictive analytics to drive business outcomes:
Fraud Detection
Many banks use AI-driven predictive analytics to identify fraudulent transactions in real-time. By analyzing patterns and anomalies in transaction data, these systems can flag suspicious activity and prevent financial losses.
Credit Scoring
Predictive analytics is also employed in credit scoring, where AI models assess the creditworthiness of borrowers. By analyzing a wide range of data points, including payment history and social behavior, lenders can make more informed lending decisions.
Investment Strategies
Investment firms leverage predictive analytics to forecast market trends and optimize their portfolios. By analyzing historical data and market signals, these firms can identify potential investment opportunities and adjust their strategies accordingly.
Conclusion
As the financial landscape continues to evolve, predictive analytics powered by AI will play a pivotal role in shaping the future of finance and banking. By implementing effective AI analytics tools, financial institutions can enhance their ability to anticipate market trends, make data-driven decisions, and ultimately drive growth. Embracing this technology is no longer an option but a necessity for organizations looking to remain competitive in an increasingly data-driven world.
Keyword: AI predictive analytics in finance