Digital Twins and AI Analytics Transform Manufacturing Efficiency
Topic: AI Analytics Tools
Industry: Manufacturing
Discover how digital twins and AI analytics are revolutionizing manufacturing with real-time monitoring predictive maintenance and process optimization
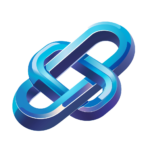
The Rise of Digital Twins: AI Analytics for Real-Time Production Monitoring
Understanding Digital Twins in Manufacturing
Digital twins represent a revolutionary advancement in the manufacturing sector, enabling organizations to create virtual replicas of physical assets, processes, or systems. This innovative technology allows manufacturers to monitor real-time performance, simulate scenarios, and predict outcomes, all of which are pivotal for enhancing efficiency and reducing downtime.
The Role of AI in Digital Twin Technology
Artificial Intelligence (AI) plays a crucial role in maximizing the potential of digital twins. By integrating AI analytics, manufacturers can harness vast amounts of data generated from their operations. This data, when analyzed, provides actionable insights that facilitate informed decision-making and strategic planning.
Key AI Implementations in Digital Twins
Implementing AI within digital twin frameworks can take several forms, including predictive maintenance, quality assurance, and process optimization. Here are a few specific applications:
1. Predictive Maintenance
AI algorithms can analyze historical data and real-time sensor inputs to predict when machinery is likely to fail. Tools such as Predictive Analytics for Manufacturing (PAM) utilize machine learning to identify patterns that precede equipment failure, enabling manufacturers to perform maintenance proactively, thereby minimizing unplanned downtime.
2. Quality Assurance
Digital twins can monitor product quality throughout the manufacturing process. AI-driven tools like IBM Watson IoT can analyze data from production lines to detect anomalies in product quality. By employing computer vision and machine learning, manufacturers can ensure that products meet quality standards before reaching the market.
3. Process Optimization
AI can also optimize production processes by simulating various operational scenarios. Tools like Siemens MindSphere allow manufacturers to create digital twins of their entire production environment. By analyzing different variables, manufacturers can identify the most efficient processes and implement changes that enhance productivity.
Examples of AI-Driven Products for Digital Twin Implementation
Several AI-driven products can be instrumental in the implementation of digital twins for real-time production monitoring:
1. Microsoft Azure Digital Twins
This platform provides a comprehensive environment for creating digital models of physical environments. Azure Digital Twins enables manufacturers to integrate AI and IoT data, allowing for real-time monitoring and analysis of production processes.
2. ANSYS Twin Builder
ANSYS Twin Builder is another powerful tool that allows engineers to create and simulate digital twins of their systems. By leveraging AI, this tool can perform predictive analyses and optimize system performance based on real-time data.
3. GE Digital’s Predix
Predix is designed specifically for industrial applications, providing a robust platform for developing digital twins. It integrates AI analytics to enhance operational efficiency and drive predictive maintenance strategies.
Conclusion
The rise of digital twins, powered by AI analytics, is transforming the manufacturing landscape. By implementing AI-driven tools and technologies, manufacturers can achieve unprecedented levels of efficiency, quality, and operational insight. As the industry continues to evolve, embracing these innovations will be essential for maintaining a competitive edge in the market.
Keyword: digital twins in manufacturing