AI Transforming Clinical Trials Reducing Costs and Timelines
Topic: AI Analytics Tools
Industry: Pharmaceuticals
Discover how AI is transforming clinical trials by reducing costs and timelines by 70% through improved patient recruitment and real-time data analysis.
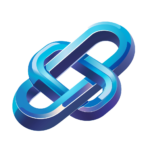
How AI is Transforming Clinical Trials: Cutting Costs and Timelines by 70%
The Current Landscape of Clinical Trials
Clinical trials are a critical component of the pharmaceutical industry, serving as the gateway for new drugs and therapies to reach the market. However, these trials are often plagued by inefficiencies, with traditional methods leading to exorbitant costs and protracted timelines. On average, a clinical trial can take over ten years to complete, with costs soaring into the billions. As the demand for faster and more cost-effective solutions intensifies, the integration of artificial intelligence (AI) analytics tools is emerging as a game changer.
AI’s Role in Clinical Trials
Artificial intelligence is revolutionizing the way clinical trials are designed, executed, and analyzed. By leveraging vast amounts of data, AI can streamline processes, enhance patient recruitment, and improve data analysis, ultimately leading to a reduction in both costs and timelines by as much as 70%.
Streamlining Patient Recruitment
One of the most significant challenges in clinical trials is patient recruitment. Traditional methods often result in lengthy delays and increased costs. AI-driven tools can analyze electronic health records (EHRs) and other data sources to identify suitable candidates for trials rapidly. For instance, IBM Watson Health utilizes advanced algorithms to match patients with appropriate clinical trials based on their medical history, demographics, and genetic information. This not only expedites recruitment but also ensures a more diverse and representative participant pool.
Optimizing Trial Design
AI can also enhance the design of clinical trials by simulating various scenarios and outcomes. Tools like Medidata’s AI platform can analyze historical trial data to predict the most effective trial design, including optimal dosages and treatment regimens. By employing predictive analytics, pharmaceutical companies can make informed decisions that reduce the risk of trial failure and shorten development timelines.
Real-time Data Monitoring and Analysis
During the trial phase, monitoring patient responses and outcomes in real-time is crucial. AI analytics tools can process data from wearable devices and mobile health applications, providing continuous insights into patient health. For example, Oracle’s Health Sciences Cloud offers AI-driven analytics that can detect anomalies and trends in real-time, allowing researchers to make timely adjustments to the trial protocol. This proactive approach can lead to faster decision-making and improved patient safety.
Examples of AI-Driven Products in Clinical Trials
1. TrialAssure
TrialAssure is an AI-driven platform that automates the management of clinical trial data. It utilizes machine learning algorithms to streamline data entry, monitoring, and reporting, significantly reducing the administrative burden on clinical research teams.
2. Antidote
Antidote is a patient recruitment platform that leverages AI to connect patients with clinical trials tailored to their specific health conditions. By simplifying the recruitment process, Antidote accelerates the timeline for trial initiation.
3. Deep 6 AI
Deep 6 AI utilizes natural language processing (NLP) to analyze unstructured data from clinical notes and EHRs. This tool helps researchers identify eligible patients for trials more efficiently, thereby cutting down the time spent on recruitment.
Implementing AI in Clinical Trials
For pharmaceutical companies looking to implement AI analytics tools in their clinical trials, the following steps can serve as a guide:
1. Assess Current Processes
Evaluate existing trial processes to identify areas where AI can add value. This may include patient recruitment, data management, or real-time monitoring.
2. Choose the Right Tools
Select AI-driven products that align with your specific needs. Consider factors such as scalability, ease of integration, and user-friendliness.
3. Train Your Team
Invest in training for your clinical research team to ensure they are equipped to leverage AI tools effectively. This may involve workshops, online courses, or partnerships with AI experts.
4. Monitor and Adapt
Continuously monitor the performance of AI tools and be open to adapting your strategies based on insights gained from data analysis.
Conclusion
The integration of AI analytics tools in clinical trials presents a transformative opportunity for the pharmaceutical industry. By streamlining processes, enhancing patient recruitment, and enabling real-time data analysis, AI has the potential to cut costs and timelines by 70%. As the industry continues to embrace these innovations, the future of clinical trials looks promising, paving the way for faster access to life-saving therapies.
Keyword: AI in clinical trials