Machine Learning in Pharmacovigilance for Enhanced Drug Safety
Topic: AI Analytics Tools
Industry: Pharmaceuticals
Discover how machine learning enhances pharmacovigilance by improving drug safety monitoring and predicting adverse reactions in the pharmaceutical industry.
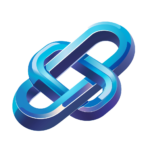
Machine Learning for Pharmacovigilance: Enhancing Drug Safety Monitoring
Introduction to Pharmacovigilance
Pharmacovigilance is a critical aspect of the pharmaceutical industry, focusing on the detection, assessment, understanding, and prevention of adverse effects or any other drug-related problems. As the global market for pharmaceuticals continues to expand, the need for robust drug safety monitoring systems has never been more pressing. In this context, machine learning (ML) and artificial intelligence (AI) are emerging as transformative technologies that can significantly enhance pharmacovigilance practices.
Implementing Machine Learning in Pharmacovigilance
The implementation of machine learning in pharmacovigilance involves leveraging algorithms that can analyze vast amounts of data to identify patterns and predict potential safety issues. By integrating AI analytics tools, pharmaceutical companies can streamline their processes, improve accuracy, and ultimately ensure patient safety.
Data Sources for Machine Learning Models
Machine learning models in pharmacovigilance can draw from various data sources, including:
- Clinical trial data
- Post-marketing surveillance reports
- Electronic health records (EHRs)
- Social media and online forums
- Scientific literature
Key Machine Learning Techniques
Several machine learning techniques can be employed in pharmacovigilance, including:
- Natural Language Processing (NLP): NLP can analyze unstructured data from reports and social media to identify adverse drug reactions (ADRs).
- Predictive Analytics: Machine learning algorithms can predict potential safety issues by analyzing historical data and identifying risk factors.
- Clustering Algorithms: These can group similar ADR reports, helping to identify trends and outliers.
Examples of AI-Driven Tools for Pharmacovigilance
Several AI-driven products and tools are currently available that can enhance drug safety monitoring:
1. IBM Watson for Drug Discovery
IBM Watson utilizes advanced AI algorithms to analyze clinical trial data, scientific literature, and real-world evidence. It can identify potential safety signals and provide insights into drug interactions, thereby aiding pharmacovigilance teams in making informed decisions.
2. Oracle’s Argus Safety
Oracle’s Argus Safety is a comprehensive pharmacovigilance solution that incorporates AI capabilities to automate case processing and signal detection. By leveraging machine learning, it enhances data analysis and improves the efficiency of safety monitoring processes.
3. BioClinica’s Safety Solutions
BioClinica offers AI-powered safety solutions that facilitate real-time monitoring of adverse events. Their tools utilize machine learning algorithms to analyze data from multiple sources, providing actionable insights for regulatory compliance and risk management.
4. Aetion Evidence Platform
The Aetion Evidence Platform uses machine learning to generate real-world evidence regarding the safety and effectiveness of drugs. This platform allows pharmaceutical companies to assess safety signals more effectively and supports regulatory submissions with robust data.
Challenges and Considerations
While the integration of machine learning in pharmacovigilance offers numerous benefits, it also presents challenges. Data privacy and security are paramount, as sensitive patient information is often involved. Additionally, ensuring the accuracy of AI models is crucial, as incorrect predictions can lead to significant consequences.
Regulatory Compliance
Pharmaceutical companies must also navigate the regulatory landscape when implementing AI-driven tools. Compliance with guidelines set forth by agencies such as the FDA and EMA is essential to maintain trust and ensure patient safety.
Conclusion
Machine learning has the potential to revolutionize pharmacovigilance by enhancing drug safety monitoring processes. By implementing AI analytics tools, pharmaceutical companies can improve their ability to detect adverse drug reactions, predict safety issues, and ultimately protect patient health. As the industry continues to evolve, embracing these technologies will be key to staying ahead in the ever-changing landscape of drug safety.
Keyword: machine learning pharmacovigilance tools