AI Tools for Accurate Demand Forecasting in Retail
Topic: AI Analytics Tools
Industry: Retail and E-commerce
Discover how AI-driven predictive analytics enhances demand forecasting in retail for improved inventory management and customer satisfaction. Unlock growth today.
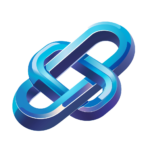
Predictive Analytics in Retail: AI Tools for Accurate Demand Forecasting
Understanding Predictive Analytics
Predictive analytics leverages historical data, statistical algorithms, and machine learning techniques to identify the likelihood of future outcomes based on historical trends. In the retail sector, this approach is invaluable for understanding consumer behavior, managing inventory, and optimizing supply chains. By predicting demand accurately, retailers can enhance customer satisfaction and drive profitability.
The Role of AI in Demand Forecasting
Artificial intelligence (AI) transforms predictive analytics by enabling more sophisticated data processing and analysis. AI tools can analyze vast amounts of data from various sources, including sales history, market trends, and social media sentiment, to provide accurate demand forecasts. This capability allows retailers to make informed decisions regarding inventory management, pricing strategies, and promotional activities.
Key Benefits of AI-Driven Demand Forecasting
- Improved Accuracy: AI algorithms can process complex datasets and identify patterns that traditional methods may overlook, leading to more precise forecasts.
- Real-Time Insights: AI tools can provide up-to-the-minute data analysis, allowing retailers to respond quickly to changing market conditions.
- Enhanced Customer Experience: By predicting demand accurately, retailers can ensure product availability, leading to higher customer satisfaction and loyalty.
AI Tools for Demand Forecasting
Several AI-driven products and tools are available to assist retailers in demand forecasting. Here are some notable examples:
1. IBM Watson Analytics
IBM Watson Analytics offers advanced data analysis capabilities, allowing retailers to visualize trends and generate forecasts based on historical sales data. Its AI algorithms can uncover insights that help retailers make strategic decisions regarding inventory and marketing.
2. Microsoft Azure Machine Learning
Microsoft Azure Machine Learning provides retailers with a robust platform to build, train, and deploy machine learning models. Retailers can use this tool to analyze customer data, predict demand fluctuations, and adjust their inventory accordingly.
3. SAP Integrated Business Planning
SAP Integrated Business Planning (IBP) combines advanced analytics with planning capabilities. It allows retailers to create demand forecasts based on various factors, including seasonal trends and promotional activities, ensuring that inventory levels align with customer demand.
4. Google Cloud AI
Google Cloud AI offers a suite of machine learning tools that can be used for predictive analytics in retail. Retailers can leverage these tools to analyze customer behavior, optimize pricing strategies, and forecast demand more accurately.
5. Demand Forecasting Solutions by Forecast Pro
Forecast Pro specializes in demand forecasting solutions that incorporate AI and machine learning. This tool enables retailers to generate reliable forecasts and improve inventory management, reducing excess stock and minimizing stockouts.
Implementing AI Tools in Retail
To effectively implement AI-driven predictive analytics tools, retailers should follow a structured approach:
1. Data Collection
Gather data from various sources, including sales history, customer behavior, and market trends. The quality and breadth of data collected will significantly impact the accuracy of forecasts.
2. Tool Selection
Choose the appropriate AI tools that align with the specific needs of the business. Factors to consider include ease of integration, scalability, and the ability to generate actionable insights.
3. Model Development
Develop machine learning models tailored to the retail environment. This may involve training models on historical data and continuously refining them based on new data inputs.
4. Continuous Monitoring
Regularly monitor the performance of predictive models and adjust them as necessary. This ensures that forecasts remain accurate as market conditions evolve.
Conclusion
As the retail landscape becomes increasingly competitive, leveraging AI-driven predictive analytics tools for demand forecasting is essential. By implementing these advanced technologies, retailers can enhance their operational efficiency, improve customer satisfaction, and ultimately drive growth. The future of retail lies in the ability to anticipate customer needs accurately, and predictive analytics powered by AI is the key to unlocking this potential.
Keyword: AI demand forecasting tools