AI for Real-Time Network Anomaly Detection and Cybersecurity
Topic: AI App Tools
Industry: Cybersecurity
Discover how AI enhances real-time network anomaly detection to protect your organization from cyber threats and maintain data integrity and operational continuity
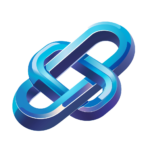
Leveraging AI for Real-Time Network Anomaly Detection
Understanding Network Anomalies
In today’s digital landscape, network anomalies pose significant threats to organizational security. These anomalies can manifest as unusual patterns in network traffic, unauthorized access attempts, or deviations from established user behavior. Identifying and mitigating these anomalies in real-time is crucial for maintaining the integrity of sensitive data and ensuring operational continuity.
The Role of Artificial Intelligence in Cybersecurity
Artificial Intelligence (AI) has emerged as a transformative force in the realm of cybersecurity. By leveraging machine learning algorithms and advanced data analytics, AI can significantly enhance the ability to detect and respond to network anomalies. Implementing AI-driven solutions enables organizations to analyze vast amounts of data in real-time, identifying threats that may go unnoticed by traditional security measures.
How AI Can Be Implemented for Anomaly Detection
To effectively implement AI for real-time network anomaly detection, organizations should consider the following strategies:
- Data Collection: Gather extensive data from various network sources, including logs, traffic patterns, and user behavior analytics.
- Machine Learning Model Training: Train machine learning models using historical data to recognize normal network behavior and identify deviations that may indicate anomalies.
- Real-Time Monitoring: Deploy AI tools that continuously monitor network activity, applying trained models to detect and alert on anomalies as they occur.
- Automated Response Mechanisms: Integrate AI with incident response protocols to enable automated actions in response to detected anomalies, reducing response time and minimizing potential damage.
Examples of AI-Driven Tools for Network Anomaly Detection
Several AI-driven tools and platforms are available that can enhance an organization’s ability to detect network anomalies in real-time:
1. Darktrace
Darktrace utilizes machine learning and AI algorithms to create a self-learning system that can identify and respond to threats in real-time. Its Enterprise Immune System mimics the human immune system, detecting anomalies based on deviations from established patterns of behavior across the network.
2. Vectra AI
Vectra AI focuses on detecting cyber threats through its Cognito platform, which analyzes network traffic and user behavior. By employing AI to prioritize alerts based on the severity of anomalies, Vectra enables security teams to focus on the most critical threats first.
3. Cisco Secure Network Analytics
Cisco’s Secure Network Analytics (formerly Stealthwatch) employs machine learning to monitor network traffic and detect anomalies. It provides visibility into user and device behavior, allowing organizations to identify potential threats and respond quickly.
4. Splunk
Splunk’s AI-powered analytics capabilities help organizations to collect and analyze machine data from various sources. Its anomaly detection features enable security teams to spot unusual patterns and potential threats in real-time.
Conclusion
As cyber threats continue to evolve, leveraging AI for real-time network anomaly detection is no longer optional; it is a necessity. By implementing AI-driven tools and strategies, organizations can enhance their security posture, reduce response times, and ultimately protect their valuable assets. The future of cybersecurity lies in the seamless integration of AI technologies that empower businesses to stay one step ahead of potential threats.
Keyword: real-time network anomaly detection