AI Demand Forecasting for Real Time Energy Management Solutions
Topic: AI App Tools
Industry: Energy and Utilities
Explore how AI-driven demand forecasting enhances energy management by improving accuracy and grid stability while optimizing resource allocation in real-time
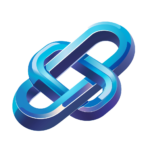
AI-Driven Demand Forecasting: Balancing the Grid in Real-Time
Understanding the Importance of Demand Forecasting in Energy Management
In the rapidly evolving landscape of energy and utilities, demand forecasting has emerged as a critical function for ensuring grid stability and operational efficiency. Accurate demand forecasting enables energy providers to anticipate consumption patterns, optimize resource allocation, and reduce operational costs. However, traditional forecasting methods often fall short in adapting to the dynamic nature of energy consumption. This is where artificial intelligence (AI) steps in, offering innovative solutions that enhance the accuracy and responsiveness of demand forecasting.
The Role of AI in Demand Forecasting
AI algorithms can analyze vast amounts of historical and real-time data, identifying patterns and trends that human analysts may overlook. By leveraging machine learning, AI-driven demand forecasting tools can continuously learn from new data inputs, improving their predictive capabilities over time. This adaptability is crucial for energy providers aiming to balance supply and demand efficiently.
Key AI Techniques for Demand Forecasting
Several AI techniques are particularly effective in demand forecasting:
- Time Series Analysis: This involves analyzing historical consumption data to predict future demand. AI models can identify seasonal trends and anomalies, providing a more nuanced understanding of energy usage patterns.
- Neural Networks: These algorithms mimic the human brain’s functioning, allowing for complex pattern recognition in large datasets. They are particularly useful for capturing non-linear relationships in energy consumption.
- Natural Language Processing (NLP): NLP can analyze unstructured data, such as customer feedback and social media sentiment, to gauge public perception and its impact on energy demand.
AI-Driven Tools for Energy Demand Forecasting
Several AI-driven tools and platforms are currently transforming demand forecasting in the energy sector. Below are some notable examples:
1. AutoGrid
AutoGrid utilizes AI to provide real-time demand forecasting and grid optimization solutions. By analyzing data from various sources, including smart meters and weather forecasts, AutoGrid’s platform helps utilities predict energy demand with remarkable accuracy. Its ability to balance supply and demand in real-time significantly enhances grid reliability.
2. Siemens’ Demand Response Management
Siemens offers a comprehensive demand response management solution that employs AI to optimize energy consumption across multiple sectors. By forecasting demand fluctuations, Siemens’ tool enables utilities to implement demand response strategies effectively, ensuring that energy supply meets real-time needs.
3. IBM Watson IoT
IBM’s Watson IoT platform integrates AI capabilities to enhance demand forecasting for energy utilities. By leveraging machine learning algorithms, Watson IoT analyzes data from connected devices and sensors, providing insights into consumption patterns and enabling proactive resource management.
4. Enel X
Enel X provides AI-driven analytics tools that help utilities forecast demand and manage energy resources efficiently. Their platform combines historical data with predictive analytics to create accurate demand models, allowing for better planning and optimization of energy distribution.
Implementing AI for Enhanced Demand Forecasting
To successfully implement AI-driven demand forecasting solutions, energy providers should consider the following steps:
- Data Collection: Gather historical and real-time data from various sources, including smart meters, weather data, and customer usage patterns.
- Choosing the Right Tools: Select AI tools that align with organizational goals and infrastructure. Evaluate options based on scalability, ease of integration, and performance.
- Continuous Learning: Implement machine learning models that improve over time by continuously learning from new data inputs.
- Collaboration: Foster collaboration between data scientists, energy analysts, and operational teams to ensure that insights from AI tools are effectively utilized in decision-making.
The Future of AI in Energy Demand Forecasting
As the energy sector continues to evolve, the integration of AI in demand forecasting will become increasingly essential. The ability to anticipate and respond to demand fluctuations in real-time not only enhances operational efficiency but also contributes to a more sustainable energy future. By leveraging AI-driven tools, energy providers can achieve a more balanced grid, ultimately benefiting consumers and the environment alike.
Conclusion
AI-driven demand forecasting represents a significant advancement in the energy and utilities sector. By embracing these technologies, organizations can enhance their forecasting accuracy, improve grid reliability, and optimize resource management. As the industry moves towards a more data-driven approach, the potential for AI to transform demand forecasting is immense, paving the way for smarter and more efficient energy systems.
Keyword: AI demand forecasting for energy