AI Tools for Predictive Maintenance in Telecom Networks
Topic: AI App Tools
Industry: Telecommunications
Discover how AI tools enhance predictive maintenance in telecom networks reducing downtime and costs while improving customer satisfaction and operational efficiency
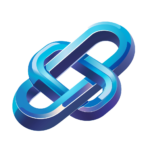
Predictive Maintenance: AI Tools Keeping Telecom Networks Running Smoothly
Understanding Predictive Maintenance in Telecommunications
Predictive maintenance is an innovative approach that leverages artificial intelligence (AI) to enhance the reliability and efficiency of telecom networks. By utilizing data analytics and machine learning algorithms, telecom operators can anticipate equipment failures before they occur, thereby minimizing downtime and maintenance costs. This proactive strategy not only improves service quality but also optimizes resource allocation.
The Role of AI in Predictive Maintenance
Artificial intelligence plays a pivotal role in transforming traditional maintenance practices into more predictive and data-driven methodologies. AI tools can analyze vast amounts of data collected from network devices, identifying patterns and anomalies that may indicate potential failures. This capability allows telecom companies to make informed decisions about maintenance schedules and resource deployment.
Key Benefits of AI-Driven Predictive Maintenance
- Reduced Downtime: By predicting equipment failures, telecom operators can schedule maintenance during off-peak hours, minimizing service interruptions.
- Cost Efficiency: Predictive maintenance reduces the need for emergency repairs and extends the lifespan of network equipment, leading to significant cost savings.
- Improved Customer Satisfaction: Enhanced network reliability translates to better service quality, resulting in higher customer satisfaction and retention rates.
AI Tools and Products for Predictive Maintenance
Several AI-driven tools and products have emerged in the telecommunications sector, enabling companies to implement predictive maintenance effectively. Here are some noteworthy examples:
1. IBM Watson IoT
IBM Watson IoT offers advanced analytics capabilities that allow telecom operators to monitor network equipment in real-time. By utilizing machine learning algorithms, the platform can identify potential issues and suggest preventive measures, ensuring seamless network operations.
2. Siemens Mindsphere
Siemens Mindsphere is a cloud-based IoT operating system that integrates AI for predictive maintenance. It enables telecom companies to analyze data from connected devices, providing insights that help in forecasting failures and optimizing maintenance schedules.
3. GE Predix
GE Predix is another powerful platform designed for asset performance management. It uses AI and machine learning to analyze operational data, allowing telecom operators to predict equipment failures and implement maintenance strategies that enhance overall network reliability.
4. Nokia AVA
Nokia AVA is a comprehensive AI-powered analytics platform tailored for the telecommunications industry. It provides predictive insights across various network layers, enabling operators to proactively manage their assets and ensure optimal performance.
Implementing AI for Predictive Maintenance
To successfully implement AI-driven predictive maintenance, telecom companies should consider the following steps:
1. Data Collection and Integration
Gathering data from various sources, including network devices, sensors, and operational systems, is crucial. Integrating this data into a centralized platform allows for comprehensive analysis.
2. Selecting the Right AI Tools
Choosing the appropriate AI tools based on specific operational needs is essential. Companies should evaluate platforms that offer scalability, flexibility, and robust analytical capabilities.
3. Training and Expertise
Investing in training and developing expertise within the organization is vital for leveraging AI tools effectively. Continuous education on AI technologies and data analysis will empower teams to make data-driven decisions.
4. Monitoring and Continuous Improvement
Once implemented, it is important to continuously monitor the performance of AI tools and refine algorithms based on feedback and new data. This iterative process ensures that predictive maintenance strategies remain effective and relevant.
Conclusion
As the telecommunications industry continues to evolve, the adoption of AI-driven predictive maintenance tools will be instrumental in maintaining operational efficiency and enhancing customer satisfaction. By leveraging advanced analytics and machine learning, telecom operators can not only predict equipment failures but also create a more resilient and reliable network infrastructure. Embracing these technologies today will pave the way for a smoother and more efficient tomorrow.
Keyword: AI predictive maintenance telecom networks