AI Dynamic Pricing in Logistics for Profit Maximization
Topic: AI App Tools
Industry: Transportation and Logistics
Discover how AI-driven dynamic pricing can maximize profits in logistics by adapting to market fluctuations and optimizing pricing strategies for success.
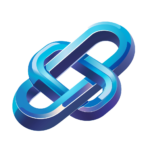
AI and Dynamic Pricing in Logistics: Maximizing Profits in Volatile Markets
Understanding Dynamic Pricing in Logistics
Dynamic pricing refers to the strategy of adjusting prices in real-time based on market demand, supply conditions, and other influencing factors. In the logistics sector, where demand can fluctuate significantly due to seasonality, economic changes, and unforeseen disruptions, implementing dynamic pricing can be a game changer for maximizing profits. Artificial intelligence (AI) plays a crucial role in enabling this strategy, providing businesses with the tools necessary to respond swiftly and accurately to market conditions.
The Role of AI in Dynamic Pricing
AI enhances dynamic pricing strategies by analyzing vast amounts of data to forecast demand, optimize pricing models, and improve decision-making processes. By leveraging machine learning algorithms, logistics companies can identify patterns in customer behavior, market trends, and competitor pricing, allowing them to set optimal prices that maximize revenue while remaining competitive.
Key AI Applications in Dynamic Pricing
Several AI applications are particularly effective in the context of dynamic pricing within logistics:
1. Predictive Analytics
Predictive analytics tools utilize historical data to forecast future demand. For example, tools like Tableau and IBM Watson Analytics can process large datasets to identify trends and predict fluctuations in demand. This information allows logistics companies to adjust their pricing strategies proactively.
2. Real-Time Data Processing
Real-time data processing tools, such as Google Cloud AI and AWS Machine Learning, enable logistics providers to monitor market conditions continuously. By analyzing data from various sources, including social media, weather forecasts, and economic indicators, companies can make informed pricing decisions on the fly.
3. Automated Pricing Solutions
Automated pricing solutions, like Pricefx and Zilliant, utilize AI algorithms to adjust prices based on predefined rules and real-time data. These platforms can help logistics companies implement dynamic pricing strategies efficiently, ensuring that prices reflect current market conditions without manual intervention.
Implementing AI-Driven Dynamic Pricing Strategies
For logistics companies looking to implement AI-driven dynamic pricing strategies, several steps can be taken:
1. Data Collection and Integration
Gathering relevant data from various sources is the first step. This includes historical sales data, competitor pricing, customer demographics, and market trends. Integrating these datasets into a centralized platform is crucial for effective analysis.
2. Choosing the Right AI Tools
Selecting the appropriate AI tools is essential for successful implementation. Companies should evaluate platforms based on their specific needs, scalability, and ease of integration with existing systems. Consideration should also be given to the level of support and expertise offered by the tool providers.
3. Continuous Monitoring and Adjustment
Once the dynamic pricing strategy is in place, continuous monitoring is vital. Companies should regularly review pricing performance and adjust algorithms as necessary. This iterative process ensures that pricing remains competitive and aligned with market conditions.
Case Studies: Success Stories in AI-Driven Dynamic Pricing
Several logistics companies have successfully implemented AI-driven dynamic pricing strategies:
1. DHL
DHL utilized AI analytics to optimize its pricing models, leading to a significant increase in profitability. By analyzing customer purchasing patterns and market trends, DHL was able to adjust its pricing dynamically, resulting in improved customer satisfaction and higher revenue.
2. FedEx
FedEx implemented machine learning algorithms to enhance its pricing strategies. By leveraging real-time data, the company could respond to market fluctuations promptly, ensuring that its pricing remained competitive while maximizing profit margins.
Conclusion
In today’s volatile markets, the integration of AI into dynamic pricing strategies is not just advantageous; it is essential for logistics companies aiming to maximize profits. By harnessing the power of AI-driven tools and applications, businesses can effectively navigate market fluctuations, optimize pricing, and ultimately enhance their bottom line. As the logistics landscape continues to evolve, embracing these technologies will be key to sustaining competitive advantage.
Keyword: AI dynamic pricing logistics